Forbes.com
This article originally appeared on Forbes.com.
Of all the people held accountable for the ethical use of data and analytics today, one group has been oddly overlooked—namely, the data scientists who built the technology.
As data scientists conceptualize, design and implement analytical tools, they make choices that require judgment and nuance. While the ethics of those choices can be tricky to parse, a few basic principles can address the challenge quite well. In fact, these principles resemble the time-honored ethics articulated more than 2,300 years ago by the Hippocratic oath, which focuses on helping and not harming the sick, on patient confidentiality, and on the importance of teaching others. That ancient Greek pledge has influenced the practice of medicine since then.
Through a similar oath for data scientists, each item would address one aspect of the judgment that data scientists must exercise every day.
I recognize that data science has material consequences for individuals and society, so no matter what project or role I pursue, I will use my skills for their well-being.
How should data scientists dedicate their time and considerable talent? Their value has soared in many employment markets, including healthcare, disaster prevention, supply chain resilience, distribution and marketing. And their selection of issues to work on will have material consequences on the broader society. Some problems have a greater impact than others, although the remuneration might not align with the relative social benefit. Each analytics expert will face trade-offs involving compensation and purpose, and collectively, their decisions will determine how quickly and effectively we address systemic problems.
I will consider the privacy, dignity and fair treatment of individuals when selecting the data permissible in a given application, putting those considerations above the model’s performance.
Many analytical models apply to situations involving both a benefit to confer and a risk to mitigate. Credit-scoring models, for instance, serve dual purposes: to help a borrower get a loan at a reasonable price and to help the lender assess the risk it’s taking. Likewise, a ride-sharing algorithm weighs risks such as passenger safety and fraud against the benefit of wider access to mobility.
Some of the data utilized to feed these models could unwittingly introduce social bias, which would prevent some people from participating in the shared activity. Many governments have prohibited financial institutions from including in their credit-worthiness algorithms factors that could create a social bias, such as race and gender. Today, as more personal data becomes available through social media and public records, data scientists should factor ethical considerations into their choices about which data sets to use and which to exclude.
A customer’s phone call history to an escort service, for instance, might be available to scientists building a model that evaluates the safety of a potential ride-sharing passenger. Yet, modern ethics would rule such data out of bounds if there is no known correlation between such calls and a person being unsafe. Using the data would violate that person’s privacy and dignity.
I have a responsibility to bring data transparency, accuracy and access to consumers, including making them aware of how their personal data is being used.
When FICO credit scores first appeared, it was difficult for U.S. consumers to get them and the underlying data unless they applied for a loan. But consumer groups began to push for the information to be more easily accessible, along with ways to correct the many data mistakes contained in the credit bureau reports. Access and the process of correcting errors have gradually improved.
Today, as companies deploy automated decision-making systems in more situations, from hiring to product offers, the experts who develop the algorithms must be transparent and rigorous about data quality—and not just in theory. Burying a user’s rights in a tiny font that no one reads simply fails any ethical test.
I will act deliberately to ensure the security of data and promote clear processes and accountability for security in my organization.
Data security now concerns corporate executives, consumers, legislators and regulators. As analytics experts pull data to power their algorithms, they have a responsibility to check and recheck that the data is secure. Companies should implement clear processes and accountability for security by hacking their own systems and regularly testing the strength of their firewalls. These processes may be difficult and time consuming, but breakdowns can lead to disaster.
I will invest my time and promote the use of resources in my organization to monitor and test data models for any unintended social harm that the modeling may cause.
Model outcomes, not just data choice and security, become increasingly important as techniques such as machine learning make models more opaque. When outsiders cannot understand what data or algorithms underpin a model, the only way to spot unintended consequences is to track and study the results.
Consider the case of Correctional Offender Management Profiling for Alternative Sanctions (COMPAS), a risk assessment software that forecasts how likely criminals are to reoffend, meaning to be arrested again by police. COMPAS guides U.S. judges as they decide, for instance, whether or not to release defendants on bail. It boasts a strong record, in that defendants assigned COMPAS’s highest risk score do eventually reoffend at a rate almost four times that of defendants assigned the lowest risk score.
While the software’s owner does not disclose the full formula, it is known that the score derives from almost 140 factors—including age and criminal history, but not race. However, a 2016 examination by ProPublica of COMPAS’s results over a period of two years in one Florida county found disturbing evidence: Among defendants who did not reoffend, COMPAS had labeled black defendants as higher risk almost twice as often as white defendants.
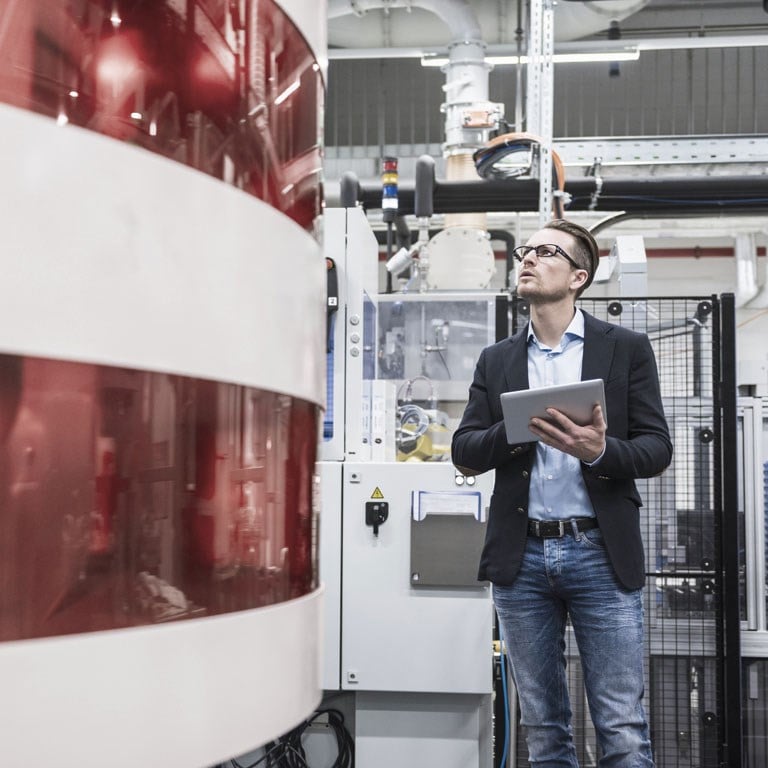
More Digital Transformation Insights
Digital transformation is a topic of rich and vital discussion in boardrooms and among executive teams around the world. Here are some insights on what it takes to lead and deliver a digital transformation.
ProPublica claimed to have evidence of the bias. COMPAS’s creators continue to argue that their product is fair, because the scores mean essentially the same thing regardless of the defendant’s race. That is, COMPAS applies the risk designation in the same way to all defendants. Both sides may be right, according to a subsequent examination by researchers from Stanford and the University of California, Berkeley. Because black defendants do get rearrested at higher rates than white defendants, a higher proportion of them will be deemed medium or high risk by the algorithm.
The crucial point is that examination of the algorithm’s outcomes by various outside groups shined a light on the use of algorithms in criminal justice. Such scrutiny after the fact forces model makers to be more cognizant of potential biases and more willing to adjust the model. Data scientists must also educate colleagues who deploy a model; without fully understanding the model, colleagues might use it inappropriately because they don’t recognize the risks.
In our data-driven world, algorithms are here to stay. But time-honored ethics endure as well. Data scientists should play an important role in defining checks and balances to create models that are both useful and fair. The Hippocratic oath has evolved over the centuries, but one concept persists: There is an art to medicine as well as science, and human traits such as sympathy also figure in its practice. We should expect no less of those who fashion products and policies out of data.
Lori Sherer is a partner with Bain & Company’s Advanced Analytics practice.