Expert Commentary
Business people who deploy advanced analytics typically face a fundamental trade-off: They must decide whether they want to use a model that predicts well or one that can be easily explained and understood. The set of tools and methods available hinges on this decision. Make the wrong choice, and they will fail in their mission.
If the goal is a model that predicts well, there are many machine learning methods to explore. These include support vector machines, neural networks, deep learning neural networks, random forests and gradient-boosted random forests. Most of these methods resemble a black box. Data goes in, and a prediction comes out. Exactly how the machine makes its prediction, however, remains a bit of a mystery. It’s difficult to observe the assumptions programmed into the machine, which variables influence the outcomes and how the variables interact.
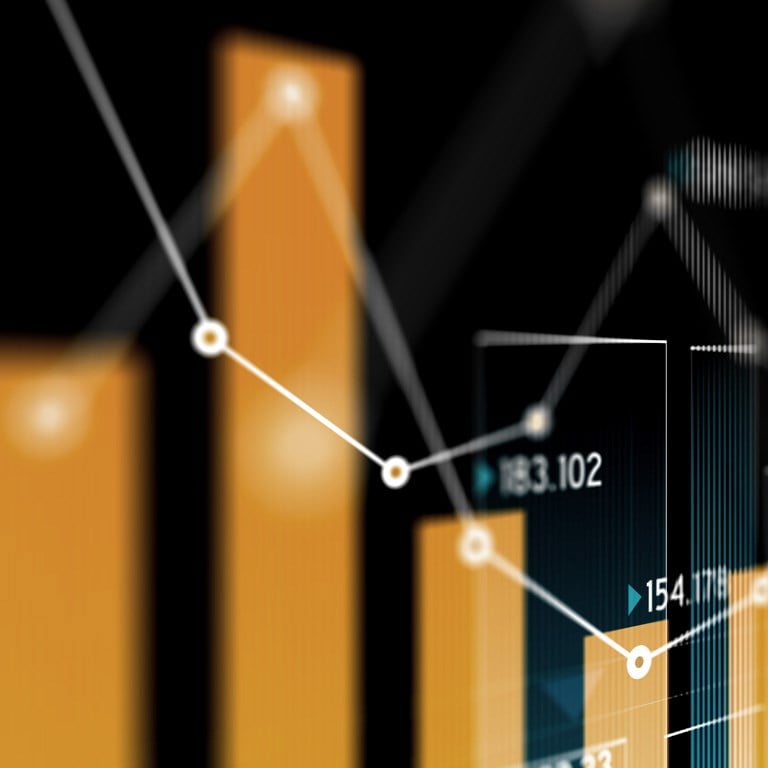
Advanced Analytics Expert Commentary
Success with advanced analytics requires both technical know-how and a thoughtful approach. In this series, Bain's experts offer practical advice on some of the most common data issues.
Consider, for example, a neural network. Analytic assumptions include the number of hidden layers, the number of nodes and the activation function. Knowing the decisions made here tells the businessperson and the analyst nothing about how the model actually works. The same applies to the variables; the analyst knows which variables were used in the model but not which variables were predictive. True, there are methods for determining which predictors are more important in these models. One method, called LIME (local interpretable model-agnostic explanations), performs individual-level sensitivity analysis to determine which predictors show the most local sensitivity in measuring the objective function. However, these methods add significant complexity and time to the analytic process and thus tend to be used infrequently.
Choosing a black-box model for its predictive powers is important if a company requires a system that works with maximum efficiency in a production environment. Logical use cases include situations of high-frequency decision making, in which the gains from improved accuracy can be quite high. Recommendation engines, predictive maintenance and high-speed trading programs all fit this model.
When a company has more prescriptive goals, we turn to different tools. The company needs to know the assumptions, variables and outcomes involved in a model so that executives can undertake specific strategies to improve performance on the variables that matter for a particular outcome. Here, traditional statistical models, such as regression and logistic regression, serve this purpose well, as do simple tree-based methods, such as CART (classification and regression tree) and CHAID (chi-squared automatic interaction detection). All of these models have the advantage of transparency. At the end of the process, we know which features were selected and the strength of each one. That helps executives make data-driven decisions.
Historically, in our work, clients have used the prescription-friendly models most often. Their goal was to explain the dynamics of a situation and inform decisions to improve a business process.
Recently, however, the balance has been shifting more toward predictive models due to two factors: complexity of the objective and complexity of the data. As an example of the former, imagine optimizing a retail assortment of 10,000 SKUs. In the case of the latter, imagine using measurements of the connections between callers or texters combined with data on cellular network performance to predict customer churn. In both cases, complexity implies that the simple answer will be insufficient. A regression model with 100 predictors may be completely transparent, yet summarizing the impact of each predictor, or collection of predictors, would be too complex for practical use.
Another consideration is that machine learning models have become more prominent in widely published studies. When managers read how these models succeed in other organizations, they get more comfortable with models that cannot easily be explained. So resistance to black boxes is falling.
One way to achieve both transparency and prediction is to start with an explainable model in order to define which actions to take. Then enhance prediction using machine learning in order to identify where to apply those actions.
Ultimately, managers will want to understand which type of model is best suited to the challenge at hand.
Paul Markowitz is a principal in Bain & Company’s Advanced Analytics practice. He is based in Boston.