Expert Commentary
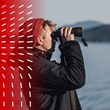
Covid-19 has challenged many forecasting processes and systems, which have failed to adapt to the huge, sudden changes in underlying demand patterns. As business as usual disappeared, self-learning models often broke down, as previously tested patterns and correlations in the past data could no longer predict what would happen in the next sales period.
During a prolonged crisis that throws demand patterns off-kilter, a more effective approach looks at forecasting holistically, including data, algorithms in the models, and process.
Adding new sources of external data, such as online searches, smartphone mobility data, and social media posts can also capture signals that are more useful for forecasting.
The same is true for the forecasting process. Businesses can update and improve their forecasts by creating scenarios, studying analogous situations such as hurricanes, and deploying human judgment. This commentary discusses ways to rebalance the level of dependence between expert judgment and models, employing the use of insights derived with dynamic approaches. It answers the question: How can we adapt forecasting models to the new normal?
When forecasting models fail
We first consider the weak spots in common models. A typical statistical forecast model will estimate future outcomes using past data. It imposes restrictions on how data patterns can be shaped, incorporating the effect of external factors and leveraging past data that may encompass or correlate with information about unknown factors that affect the demand or sales trend.
Typically, businesses use these models to forecast demand. Such models are likely to fail under sudden changing conditions, which come in two flavors:
- A shock to demand that causes a sudden spike or plunge from the baseline level. The shock is unrelated to the inputs included in the model, including price, marketing mix, or other explanatory variables. It may be permanent, temporary, or evolving, such as sudden hoarding early in a pandemic, which then eases off. This is captured by the local level component of a model.
- A change in demand dynamics. The change could be in the nature or degree of the relationship between demand and its drivers. For instance, consumers’ expected behavior around price or promotion may change suddenly in response to a new situation. That would change demand for a quantity of a given product. These changes are captured by the external factor component of the model.
Both types of change may take place simultaneously and suddenly, which can make it even more complicated for a model to correctly attribute the effects taking place, or to accurately forecast demand.
Fixing the models
Once we understand these two situations, we can revisit existing models to gauge their limitations on coping with the new environment. We can then replace them with more appropriate model choices or improve them to better deal with the changes.
To illustrate, consider three popular forecasting models: Autoregressive Integrated Moving Average with Explanatory Variable (ARIMAX), Static Bayesian Structural Time Series (BSTS), which includes popular implementations such as Facebook’s Prophet, and Dynamic BSTS (see Figure 1). The three models share some traits on how they use information, but have different performances due to their structural differences.
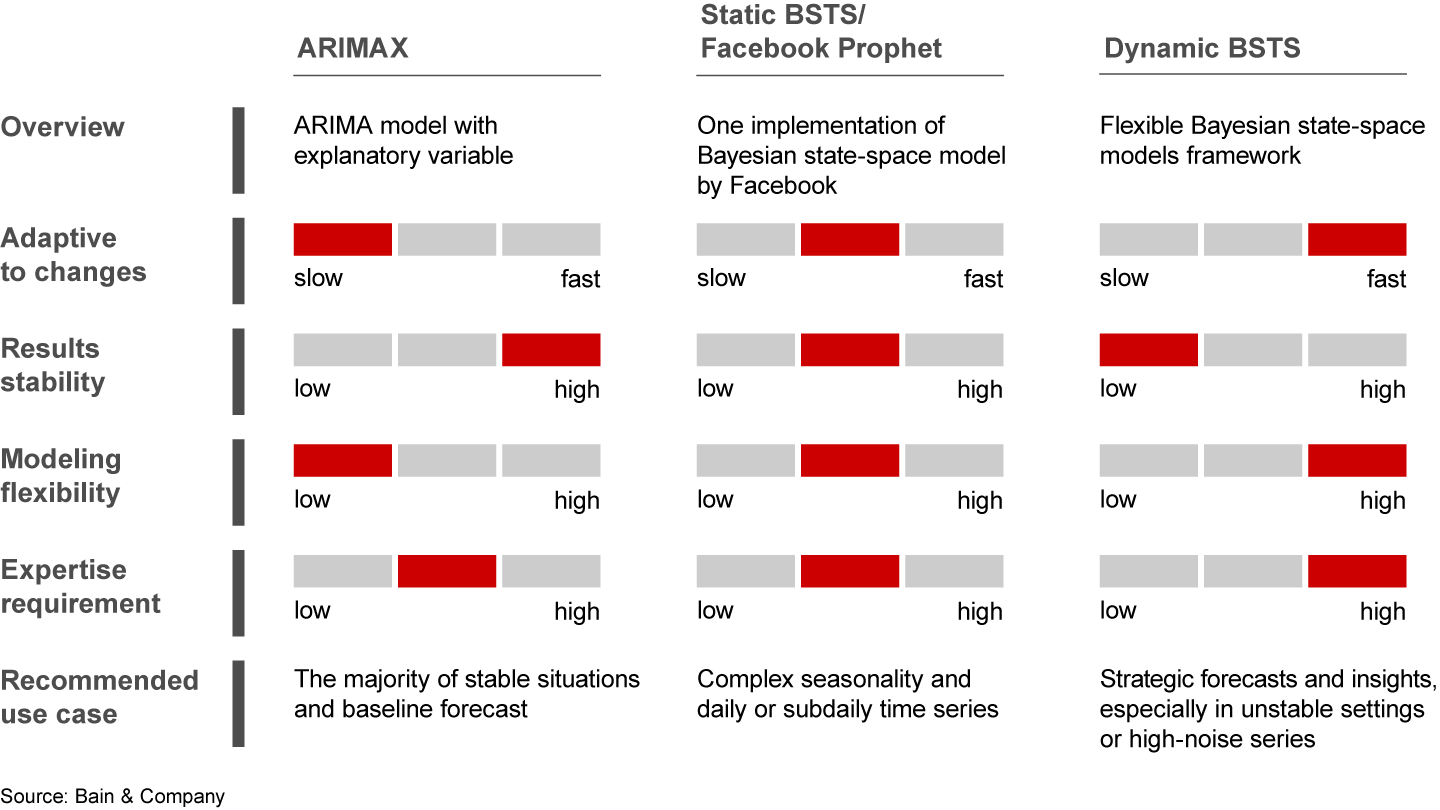
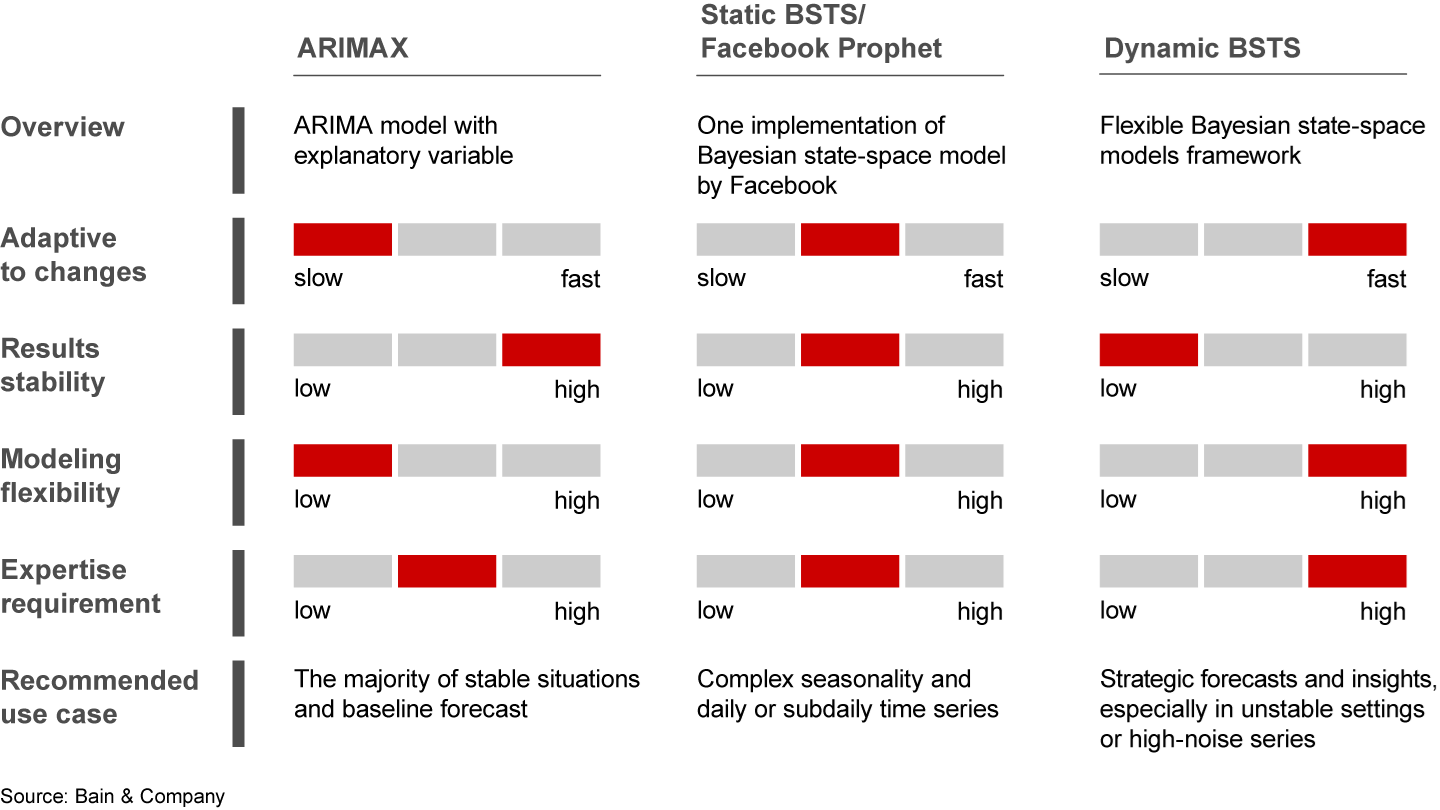
One difference lies in the “static” vs. “dynamic” concept. The Dynamic BSTS includes a dynamic regression or time-varying parameters component, which represents our concept of a dynamic model. Dynamic models allow for a reestimation of parameters through time efficiently and flexibly, which helps identify changes between demand and its drivers over time.
By contrast, the two other models are static, in that they make a single estimate of each parameter for the full time frame, which might produce more stable results.
In general, there is a trade-off between flexibility in structure along with stability and uncertainty for any statistical or machine-learning model. There will be times where a more adaptable approach will accommodate unknown or undetected changes, and other times where a more stable approach will yield a more consistent and accurate output. In practice, most forecasting models used for operations are static models.
For static algorithms, introducing additional external data may help anticipate the shock to demand dynamics; foot traffic data, for example, would capture a drop in out-of-home consumption. However, static algorithms would not anticipate a change in the demand dynamics, such as a drop in a discount’s effectiveness to increase sales.
To adapt our models to the changes, there are several options, depending on the situation, data, and insights at hand:
- Incorporate more data, which may help alleviate some effects that are distorting the relationships of inputs and outputs.
- Shorten the estimation windows for the models, which would work if the changes in dynamics are smoothly trending over time, not suddenly lurching.
- Incorporate features and insights generated by dynamic estimation. If we detect sudden breaks in the input-output relationships of the dynamic estimations, we could incorporate these into our model by creating binary interactions or other new or modified features in the model.
- Switch to better-suited models to accommodate dynamic changes, such as regime-switching models like STAR, or Dynamic BSTS itself. When used carefully, these can improve forecast accuracy and provide deeper insight.
Dynamic approaches bridge the gap
To illustrate a potential use case for Dynamic BSTS, consider unemployment claims data through the pandemic. We built a model to predict unemployment claims based on certain online keyword searches, and explored how these become more or less relevant over time, using Dynamic BSTS. Later, we used the learnings to build binary signals into our static models of when a keyword’s effect may be changing, and reestimated these models to see how their predictive performance changes.
We analyzed a sample output of the Dynamic BSTS model’s estimation of the changing relationship of unemployment claims with its drivers (see Figure 2). The red line in the figure shows at what level the estimation with a static model would be. The dark shadowed series is the level (with uncertainty intervals) at which the dynamic estimation places the effect through time. The yellow line shows the null or zero effect. The shadowed series shows that, by the end of the time series, some drivers that were not relevant before, now appear to be; and particularly by 2020, sudden changes take place in the keyword relevance effects. If we used a static model, we would end up missing these recent changes, as the overreliance on past information prevents the model from adapting.
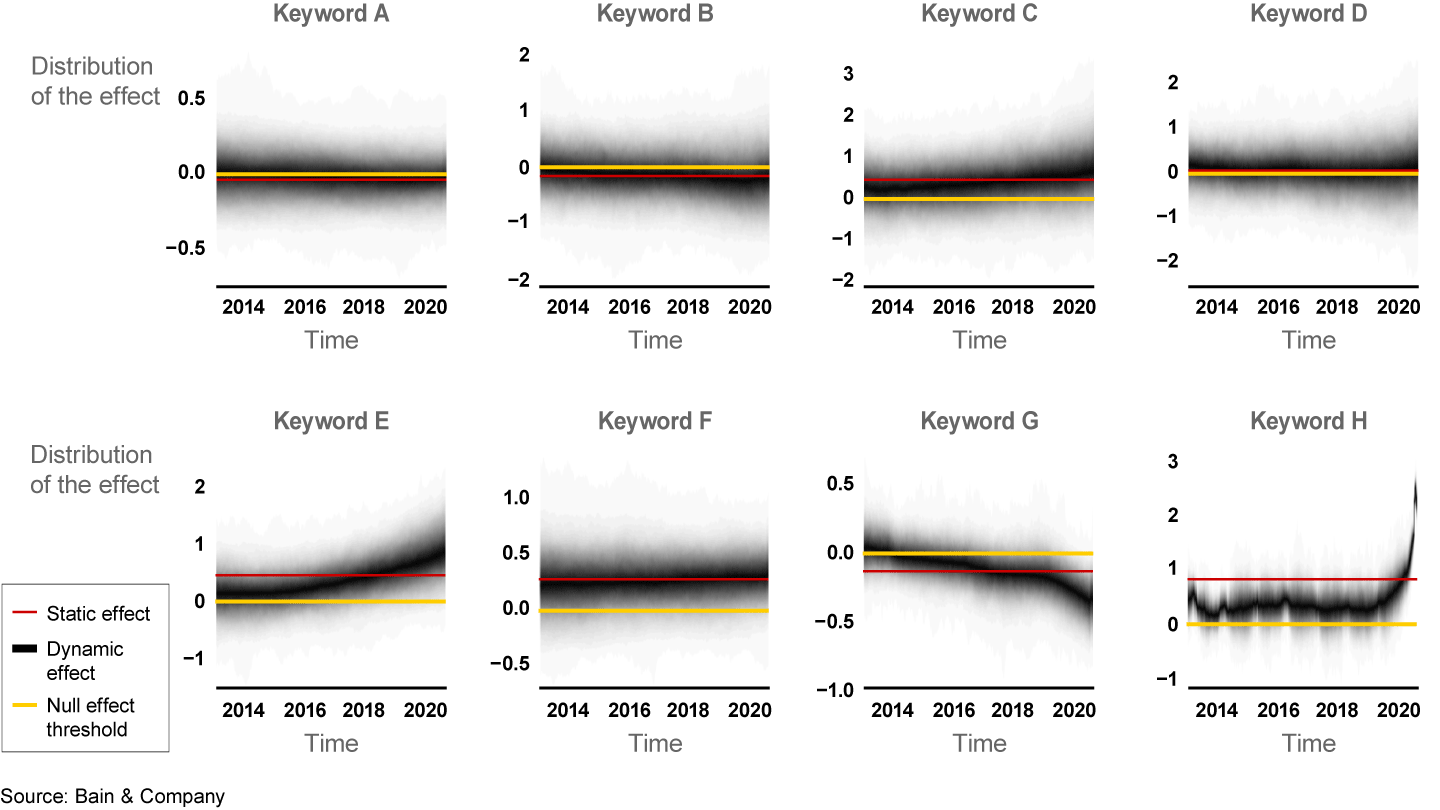
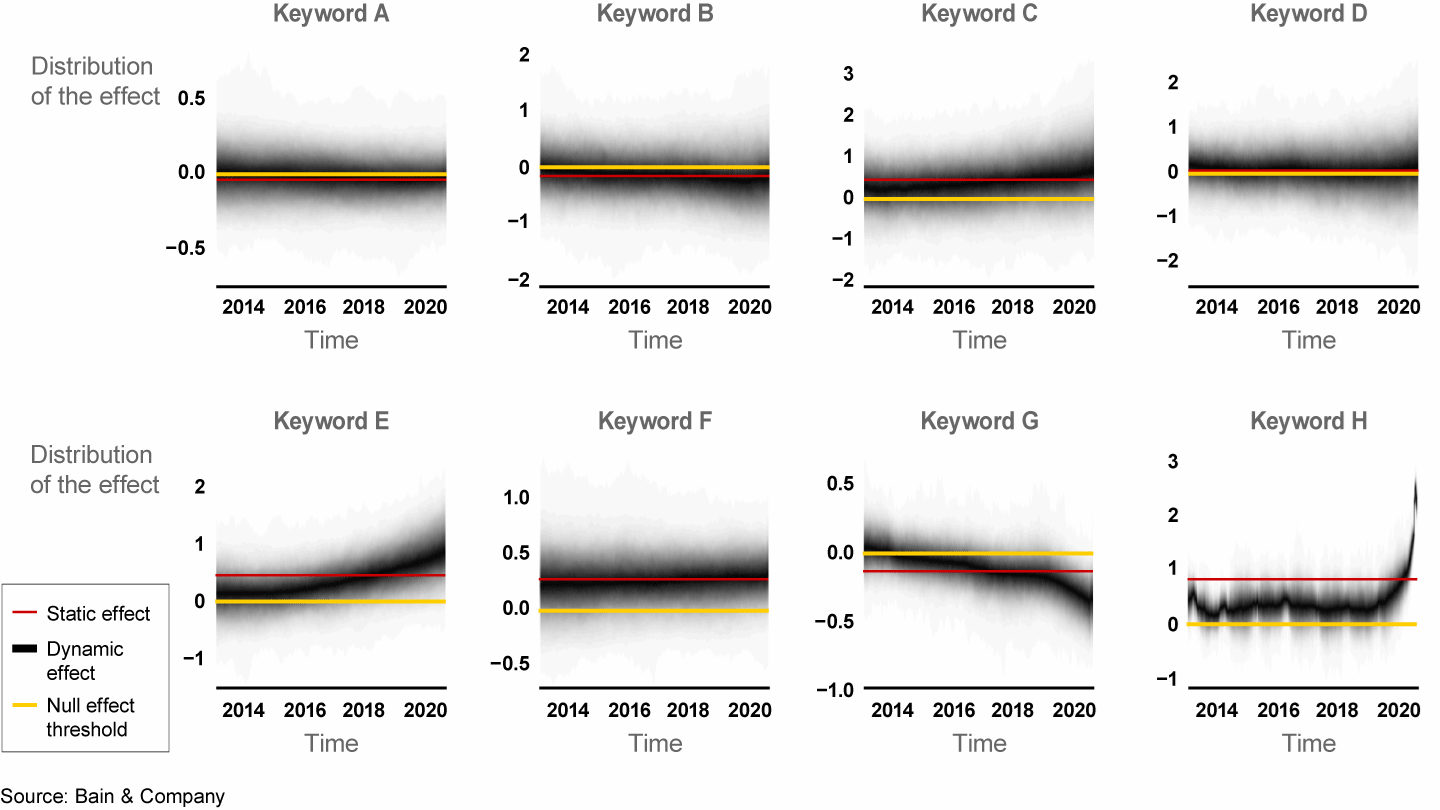
For instance, we saw that keyword H (a one-week lag of “unemployment office” searches) becomes far more relevant by the end of the estimation period and captures the sudden change in search dynamics due to the pandemic, along with other search keywords that also change behavior over time.
We could rely on the estimated model by Dynamic BSTS to make forecasts. However, when a company prefers more stable results or already has a static forecasting model in place, we can combine the strengths of both approaches: one to gather insight, and the other to model in a stable fashion. To do so, we flag within the static model any potential changes that may occur and let the estimation validate whether such changes are significant (see Figure 3).

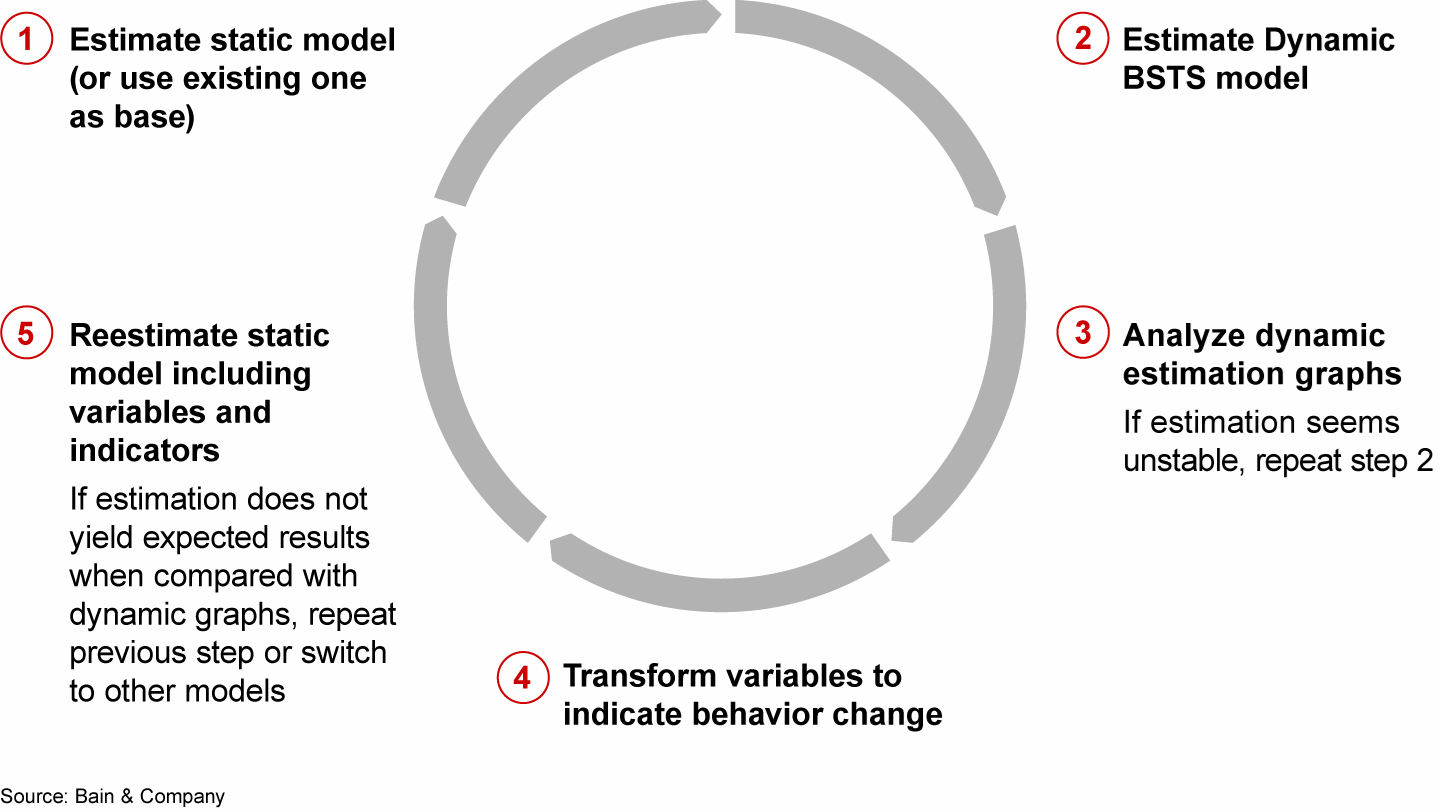
The analyst in charge can validate BSTS insights with expert judgment or more causal research before incorporating them into the model. Dynamic BSTS or related tools prove useful to generate exploratory insights and help support prior theories. The models’ forecasting performance generally benefits from this approach.
We compared the forecast of static models ARIMAX and Prophet updated using the previous insights from Dynamic BSTS (blue line) with the same models not using these insights (grey line) (see Figure 4). One can see the differences in forecasting performance of the models by using this methodology. The dynamic model captures the changes in search term relevance from Figure 2 and helps the static models reflect the new dynamics of the series much better after applying the methodology. The improvement for the Prophet algorithm is the highest, partly due to the algorithm’s flexible trend estimation method.
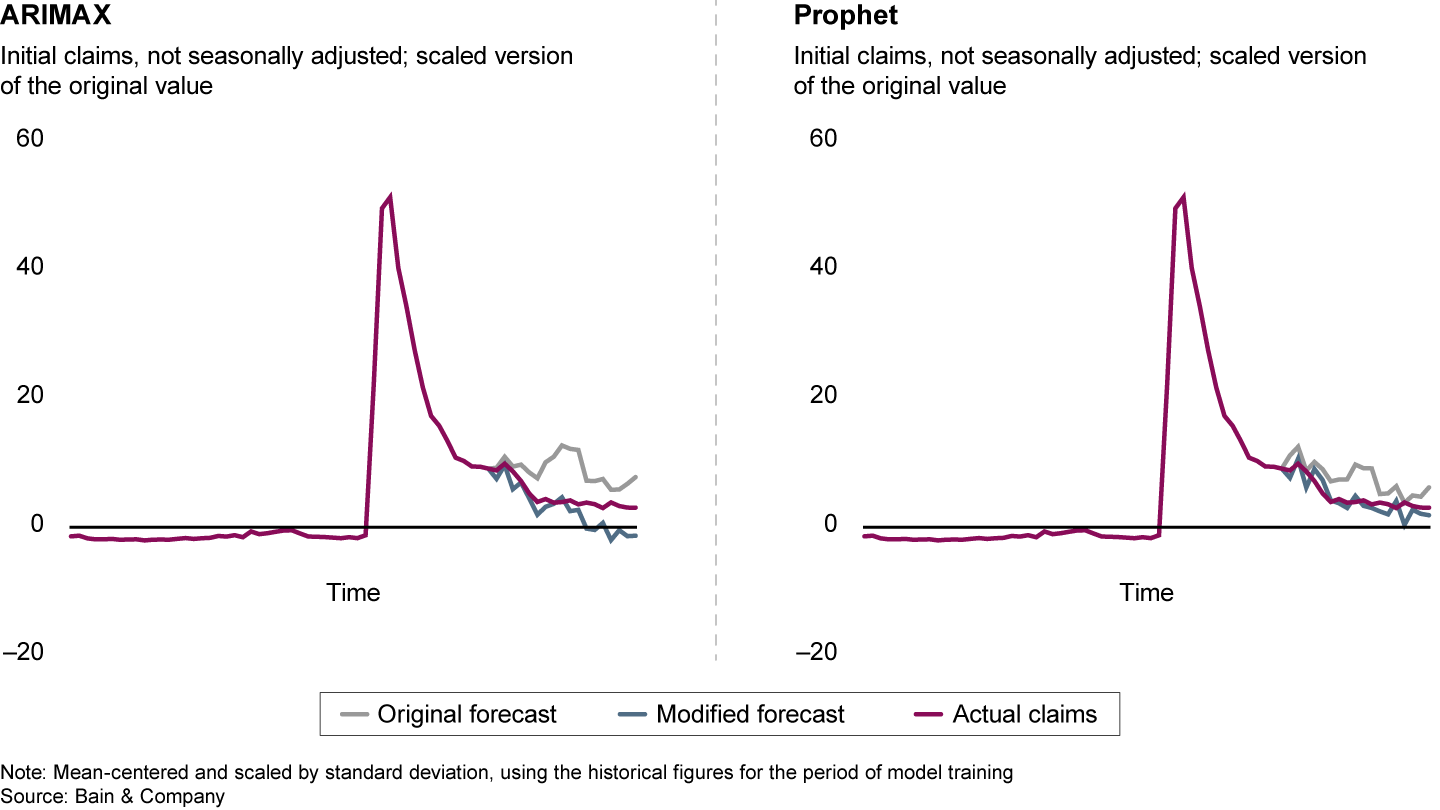
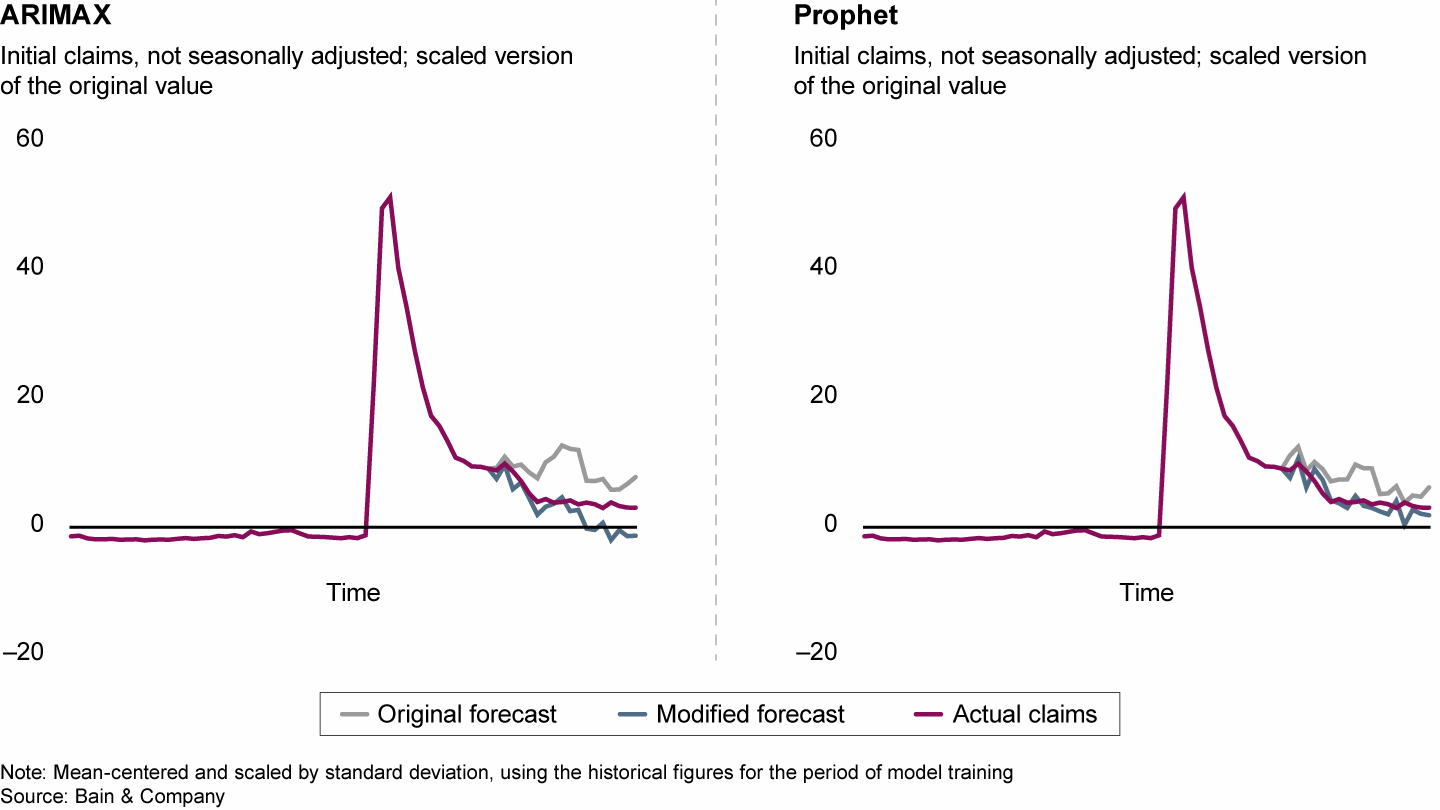
Two key points emerge from this experience:
- A dynamic approach can better capture insights from changing environments and will yield better performance in these situations.
- Previous modeling approaches and accuracy can be improved by fine-tuning through insights derived from a dynamic approach.
This is especially important when dealing with sudden changes such as Covid-19 or other sudden disruptions to a system’s functioning.
Fit for purpose
“All models are wrong, but some are useful” is a familiar aphorism in the field of analytics. To keep our models useful, we must choose the model that best fits the particular needs, and keep monitoring the effectiveness of the forecast. Existing forecast models may need to be revisited, and the use of a tool like Dynamic BSTS can help companies gain insights about underlying changes, in order to learn how to best reestimate the existing models.
Keep in mind that modeling is just one component within a forecasting system. Business leaders should assess their forecasting process holistically, including data sources, model, platform, process, feedback loop, and targeted experiments, focusing on adjustments to the components that will yield the most business value.