Report

This article is Chapter 5 of Bain’s global Technology Report 2020. Explore the contents of the report here or download the PDF to read the full report.
Our podcasts, business literature and public earnings calls confidently espouse the criticality of data, machine learning and artificial intelligence (AI) to our future. AI and machine learning (ML) companies took in nearly one-fifth of all US-based venture funding in the first half of 2020. Google and many other technology leaders are now “AI-first” companies; cloud and mobile have taken a back seat.
It’s clear that data, machine learning and AI will play a fundamental role in the technology industry’s growth and evolution in the coming years. Every business will be affected in some way by these technologies. Now, the question for executives is, “What should I do about it?”
Today’s leading AI companies recognize that data and talent are the engines of AI growth, and are investing heavily in both. The leaders are also finding new and creative ways to put AI technologies at the core of their products. These companies’ strategies hold lessons for all business executives trying to figure out how they can capitalize on these critical technologies.
A good place to start is by defining the terms “AI” and “machine learning,” how they’re being used, and what they can ultimately deliver. Our definition is intentionally broad to emphasize the intent, as opposed to any specific tool or model. Machine learning refers to techniques for developing computer algorithms that learn from data instead of being specified explicitly by programmers. Artificial intelligence uses ML and other techniques to enable programs to behave autonomously and provide human-like responses.
Coined in 1955, the term "artificial intelligence" encompasses a range of analytical and reasoning computing techniques. These started with sets of rules, called expert systems, and have evolved into complex, many-layered neural networks that train themselves using thousands of machines in cloud data centers. Like other recent technology advances, the growth of AI and ML has been spurred over the last 10-plus years by the dramatic reduction in the cost of storage and computing power driven by Moore’s Law; the proliferation of data generated by the Internet and mobile devices; and the wide availability of data and ML tools through cloud platforms and open source.
What can we learn from the early successful users of AI and ML? The key insight is they’ve engineered their businesses and products with feedback loops that capture valuable user data, which is used to build intelligent ML models, which in turn power differentiated services that increase customer stickiness and result in further data capture. LinkedIn and Netflix recommendations are good examples. Their device and web user interfaces generate data on user engagement. This enables Netflix to make personalized movie and show recommendations, and LinkedIn to provide personalized newsfeeds and recommend professionals with whom to connect.
Consumer web companies, cloud service providers and software-as-a-service businesses were the early movers, but the transition to AI-enabled businesses is coming to all technology firms. However, some are better positioned than others. Leading companies recognize that AI product development is constrained by the available talent, and they’re quickly scooping it up. Across the technology stack, the top-performing companies as measured by revenue growth are investing heavily to expand their AI workforces. In many technology segments, these leaders have two to three times more AI talent on staff than the rest of the field (see Figure 1).
Leading companies are investing heavily in AI talent
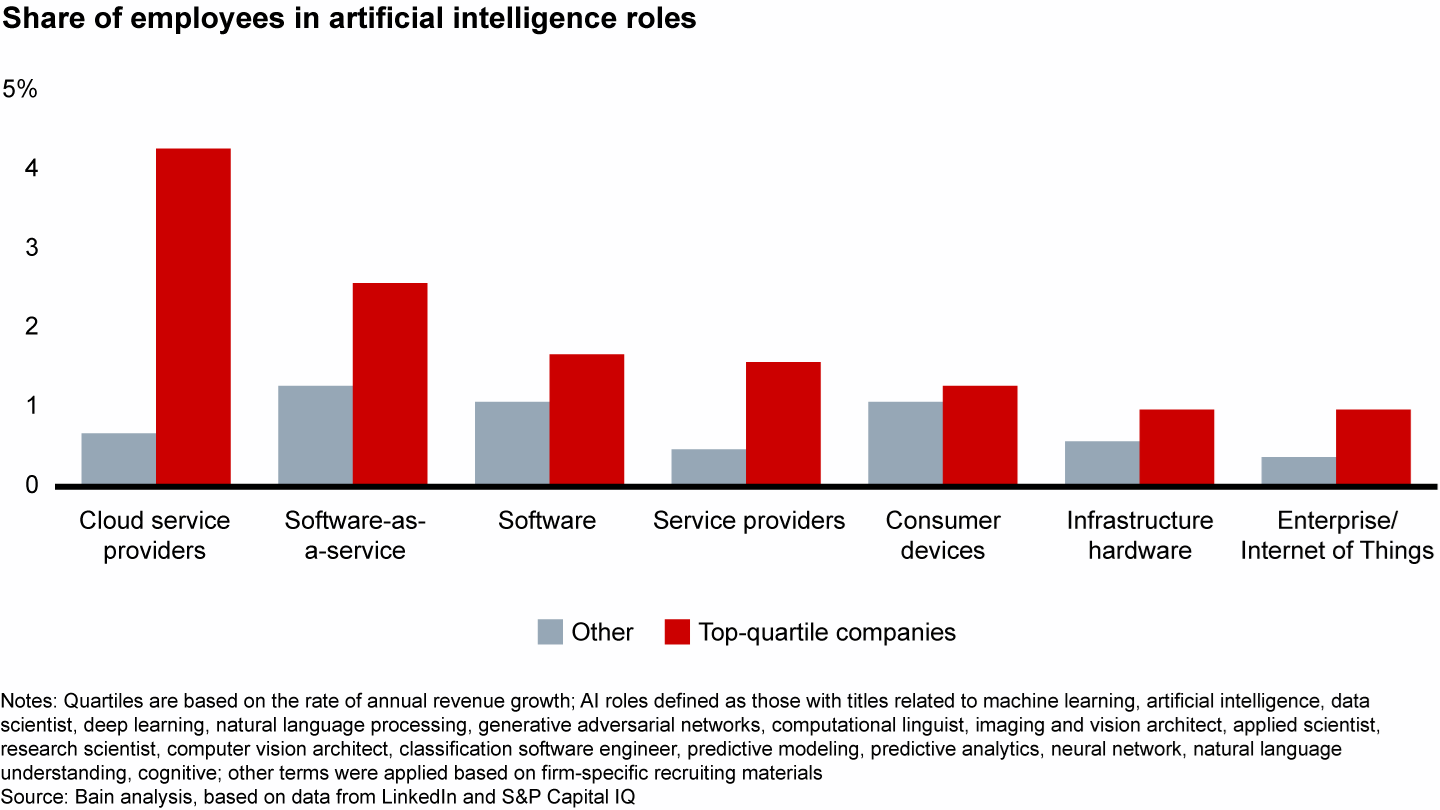
What does that mean? The leaders’ talent advantage could help them develop more innovative AI products and capture a larger share of this critical market.
AI opportunities
Leaders in the next wave of AI adoption view data, ML and AI as critical to the future of their businesses. These companies are redefining their products by infusing them with these technologies in three primary ways.
Adding features. Companies are using AI and ML to make their products smarter, more automated and ultimately more productive for customers. Since launching its Einstein tool in 2016, Salesforce has embedded AI capabilities across its primary offerings: Sales Cloud, Marketing Cloud and App Cloud. These applications now bring automation and increased intelligence to around 20 functions, including customer lead scoring, sales forecasting, language and sentiment analysis of customer communications, customer churn predictions and next-best-action recommendations.
Salesforce’s executives recognized good data—and good data management—must underpin all of this, so they acquired Krux in 2016. This data management platform interacts with more than 3 billion browsers and devices, supports more than 200 billion data collection events and processes more than 5 billion customer relationship management records. The platform’s tools unify third-party data with first-party data to improve the ML models that support customers’ applications.
Creating services. AI is affecting industries that traditionally haven’t relied much on technology. Compass, a home-brokerage start-up backed by SoftBank, hired the former chief technology officer of AI at Microsoft, Joseph Sirosh, in 2018. As CTO, Sirosh has helped Compass develop AI-powered services based on the extensive data being collected in the company’s core real estate product lines.
For example, Compass offers a “Concierge” service that recommends and fronts the cost of home improvement services such as staging, flooring and painting. Based on before-and-after photographs of tons of properties and extensive transaction data, Compass is using AI to help sellers visualize potential home improvement investments and make better-informed decisions that will maximize sale value. By the end of 2019, nearly 20% of active Compass listings included this value-added service; Compass takes a fee based on results. AI-enabled services like these have helped Compass become one of the top brokerages in the US by sales volume, according to Real Trends.
Building platforms that democratize AI. The most powerful early influencers of the AI transition are developers of cloud tools and services, including US- and China-based companies such as Google, Microsoft, Amazon, Facebook, Alibaba, Baidu and Tencent. Many of these companies saw opportunities to help their customers more effectively organize, analyze and interpret the large volume of data they were collecting and processing in the cloud (see Figure 2).
Cloud service providers are significantly expanding their AI tools and services
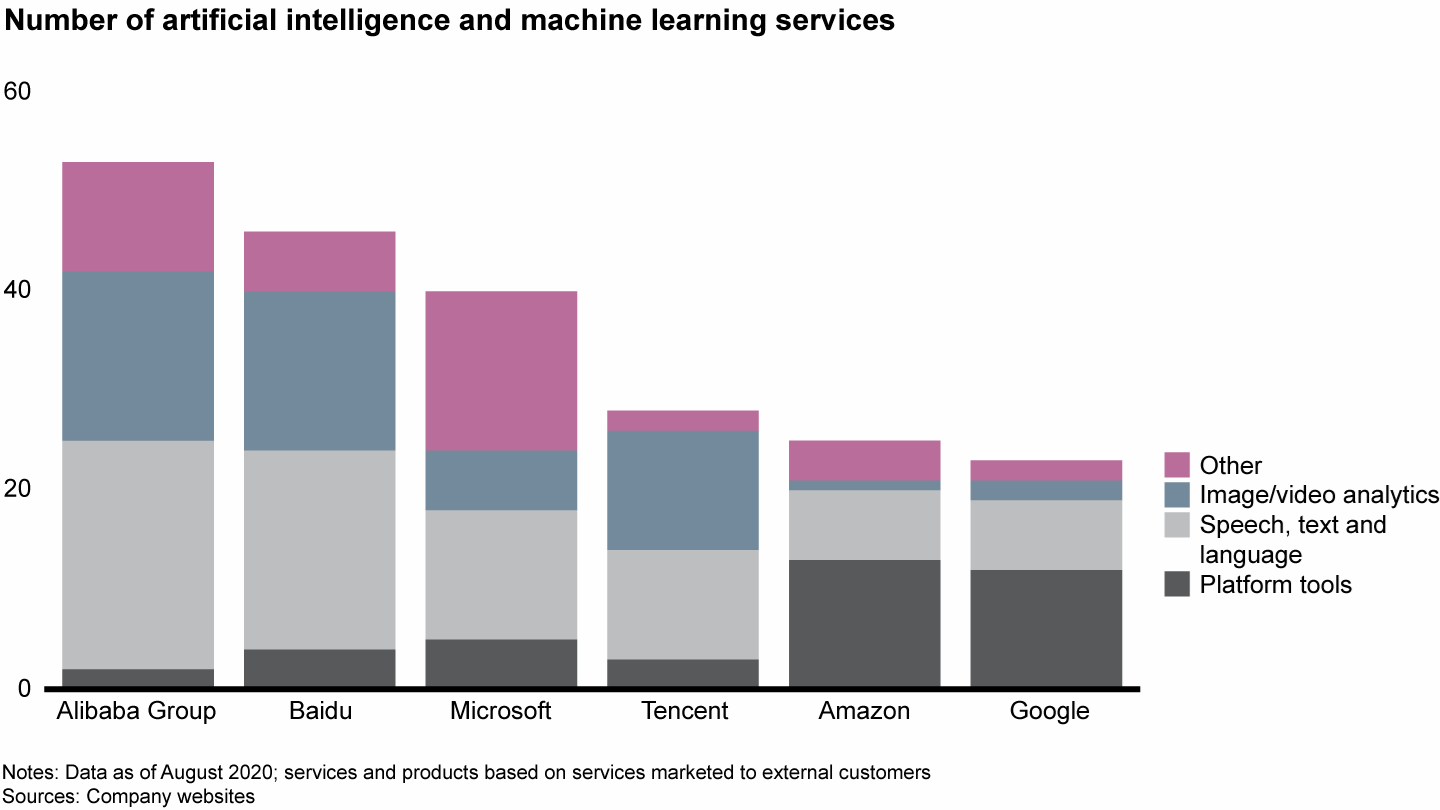
Baidu took advantage of its search engine’s leading market position in China to become the preeminent provider of ML tools in the Chinese cloud market. Based on search data from hundreds of millions of consumers, Baidu developed its Baidu Zhishu index to analyze trending interests across different user segments, geographies, topics and media, including text, images and videos. These large data sets, and the associated ML tools, were embedded into the heart of Baidu’s cloud offering and deep learning platform, PaddlePaddle. They’ve propelled the popularity of Baidu’s cloud platform among AI and ML developers.
Other cloud leaders have taken a similar approach, including Amazon, which has created an AI flywheel with its Alexa products. More data helps improve the products, which wins more customers and attracts more talent (both internal and external) to the company’s AI platform. This feeds additional advances in AI technologies and better products—and the cycle continues.
The AI journey
Now that these technologies are maturing, a lot more companies are expected to weave intelligent capabilities into their products. But the technology industry is still learning how to get the most out of AI and ML. They’re complex, and a lot can go wrong on a product’s journey from idea to development to the market. Learning from the early adopters, companies can maximize their chances of success by taking the following steps.
Build a differentiated data asset. The use of 21st century technology, computer science and applied mathematics to create personalized and automated products is an exciting topic in the boardroom. Data governance usually inspires less enthusiasm among the C-suite. Yet in the increasingly competitive AI battleground, more and better data beats better algorithms.
That’s why leading companies take an exacting approach to managing their data assets. They also make investments to enrich the value of their proprietary data assets. Web crawling, crowdsourcing, computer vision, sensors and partnership deals can all provide a significant boost to data assets.
Executive teams investing in AI should remember this important trend: the growing sensitivity around data ownership, usage and privacy. Europe has been at the forefront of regulations regarding privacy and transparency, and strong regulations are emerging across the globe in national and regional markets such as India and California.
Amid that backdrop, companies such as Google, Apple and Microsoft have developed more advanced differential privacy and homomorphic encryption techniques, and these are reaching the mainstream. The US Census Bureau now plans to release data using some of the latest privacy technologies. And healthcare groups fighting the Covid-19 pandemic are experimenting with federated learning and other privacy techniques to more securely share sensitive data.
Develop critical skills and processes. Most technology companies recognize that data scientists are a necessary component of bringing intelligence to their products. But some overlook the fact that many other roles and skills are required to support the delivery of AI and ML features. These include data and ML product management and design; data stewardship, contract negotiation and sourcing; data labeling operations; and ML system engineering and technology operations.
Developing these new capabilities can be challenging. Product designers and data scientists frequently appear to hail from different planets, and product management is still an emerging discipline at many technology companies. Top-performing companies ensure these new teams have the resources and support they need to excel.
In addition, these new capabilities require new processes that will add complexity to an organization. Google has referred to ML as “the high-interest credit card of technical debt.” The company has documented 28 separate tests that can be applied to any ML model that it uses. This is more complicated and rigorous than typical software product development processes, but while ML is more complex, the potential return on investment can be much bigger.
Redesign product workflows with data, ML and AI in mind. The most successful leadership teams think through the opportunities to use and capture data at every stage of their customers’ journey. These leaders also keep their fingers on the pulse of new battlegrounds for value-added intelligent assistant services that they can layer on top of today’s simpler product workflows. A well-known example: Google Maps has evolved from a simple query interface for locations to a context-sensitive intelligent assistant that has become an essential tool for many.
Participate in fast-moving technology ecosystems. ML and data management technologies are developed as open-source projects more often than in other software domains. Some ML and data management open-source projects have a single, large corporate backer, such as Google’s TensorFlow and Facebook’s PyTorch. Some have multiple large backers: Presto is actively developed by Facebook, Uber and Netflix, among others. And other projects have influential academic stakeholders. Projects such as Jupyter, Apache Spark and Ray, for example, all have significant ties to the University of California, Berkeley. Winning the technology, data and talent race will usually involve a mix of partnerships, strategic participation in open-source initiatives, mergers and acquisitions, and organic product development.
Leadership required
While the possibilities of AI and ML technology are exciting, organizations must invest in the underlying data, skills, processes, product designs and partnerships necessary for success. It’s tempting to assume that AI can also address some of these foundational challenges and pull itself up by its bootstraps, but in reality, human leadership and guidance will remain essential. Executives will need to apply their best judgment in placing the right AI and ML bets to navigate complex uncertainties around customer adoption, technology capabilities and ecosystem dynamics. To quote Gerd Gigerenzer, an expert in decision making: "If risks are known, good decisions require logic and statistical thinking. If some risks are unknown, good decisions also require intuition and smart rules of thumb.”