Brief
Big Data and advanced analytics have begun to yield big payoffs for a few insurance companies. And insurers globally plan to spend more on it over the next three to five years, having seen the early successes of companies such as Progressive in personal property/casualty (P&C) lines and AIG in commercial lines. Annual spending growth on Big Data analytics will reach 24% in life and 27% in P&C on average, according to the 70 insurers surveyed for Bain’s benchmarking database in 2015. Opportunities expand every day with the proliferation of new sources, including sensors, Web chat logs and videos, as well as the 195,000 datasets that US government agencies have made publicly available.
Yet most insurers have barely scratched the surface. Roughly one in three life insurers and one in five P&C insurers do not apply Big Data advanced analytics for any function, according to Bain’s benchmarking survey. On average, insurers apply Big Data to approximately two functions (see Figure 1), and many companies do not have a solid plan to wring value out of the data. Discussions tend to hover on data management issues and technology investment decisions, rather than focusing on the more important question of exactly how to derive wisdom from data in order to make better decisions.
For the firms that dig deeper, substantial benefits accrue. For example, Big Data can improve decisions in the following three areas:
A better customer experience: A life insurer wanted to reduce the cost and hassle of expensive blood tests required for prospective new customers. It found the right external health data to combine with the prospects’ answers on applications, and built an algorithm to predict which prospects would qualify without the test. The model allowed the company to eliminate blood tests for 30% of applicants.
Innovation: Some insurance companies are using analytics to create innovative new products or expand underinsured markets. Automakers, for instance, have a parts warranty exposure of more than $60 billion per year, but they lack the capability to aggregate and analyze their claims data. We Predict, a UK-based company, built an analytics engine that accurately predicts parts failure, which it now licenses to the industry. This engine helps to improve supply chain efficiency (getting the right parts to the right dealer at the right time), reduce dealer fraud (detecting when repair rates are higher than normal, and actively intervening) and raise consumer advocacy (by anticipating problems before they occur and notifying loyal customers in advance).
Underwriting and claims: At a commercial lines carrier, underwriting due diligence took up to nine months, with on-site inspections of scores of properties owned by any large business applying for coverage. The carrier decided to review its own database of clients to uncover best safety practices and then check US federal data on safety violations as a way to screen prospective clients. While the analytics have helped the carrier reduce expensive initial site inspections by engineers, the real value lies in avoiding signing on a client with a high probability of a $100 million accident down the road.
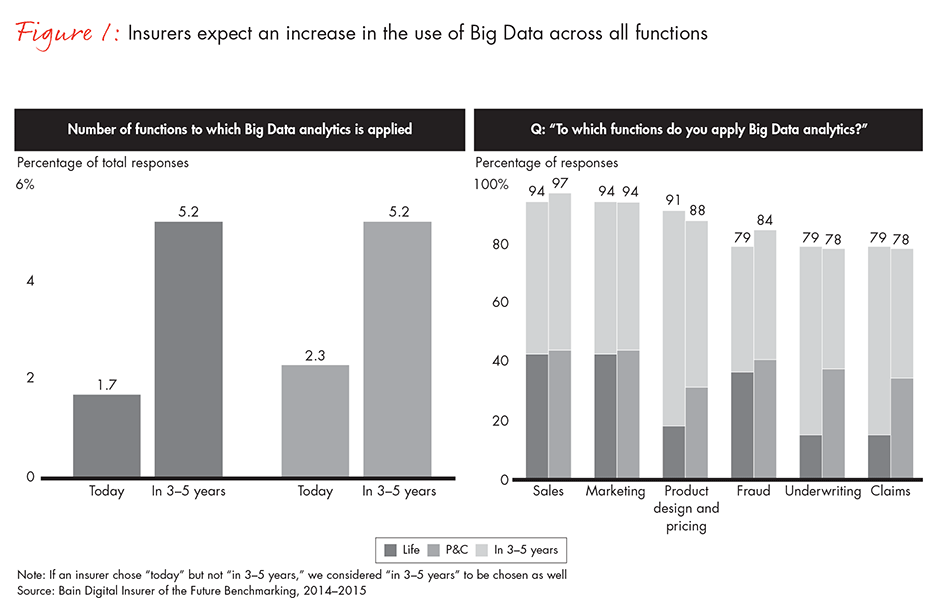
Turning to claims, Santam in South Africa wanted to reduce the fraud rate of its medical claims. Working with IBM, the company decided to analyze three years of customer data to hypothesize where fraud was most likely to occur. It then established a set of rules on claim type, amount and other red flags to segment incoming claims and take appropriate action. Claims data, combined with pathology results and customer questionnaires, has helped Santam forecast and prevent further health risks. The initiative saved $2.4 million in the first four months, and it has given Santam the ability to accelerate half of processed claims by putting them to straight-through processing. (As a side benefit, the initiative identified a motor insurance fraud syndicate in the first month.)
Start small and scale up
To get more familiar with Big Data analytics, insurers should start with small steps. They should choose a case, hypothesize what data will correlate with the behavior in question, obtain easily accessed data sets and begin modeling to see what develops. They can then build their capabilities so as to use analytics to their full potential. By applying Big Data in the right places and the right ways, insurers can develop insights that are difficult to replicate and eventually build a competitive advantage.
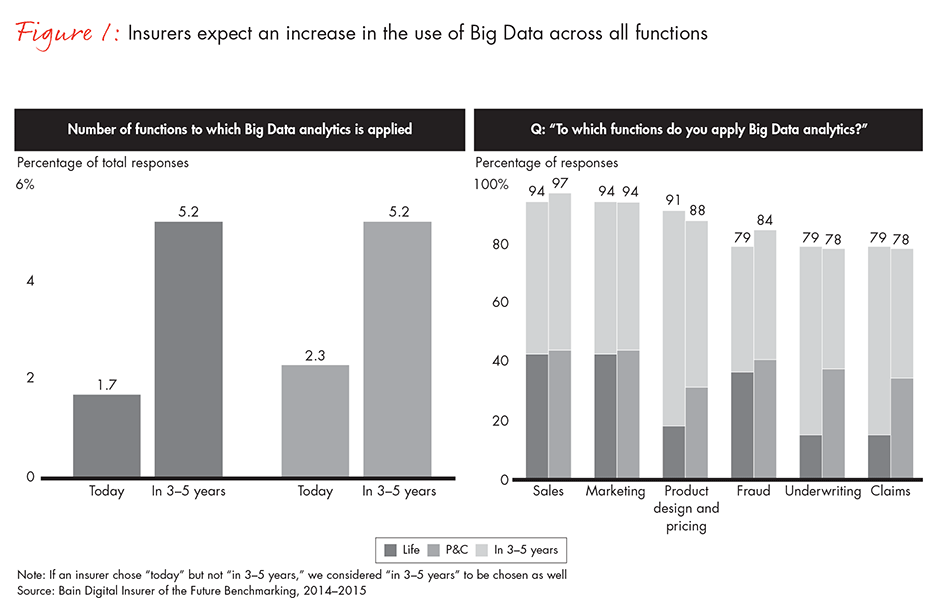