Expert Commentary
Many companies use in-market tests to find out if new variations can significantly strengthen a current practice, whether it’s a call-center script, promotion, store layout or other feature in need of improvement.
While marketers focus on properly designing new testing variations, they tend to pay less attention to selecting the sample audience. That’s shortsighted, as sample selection is critical for in-market tests with a predefined target audience. Whether it’s a simple A/B test or a complicated multivariate test, success stems in part from dividing the available target audience into multiple look-alike testing groups. The goal is to confidently attribute any significant differences in key performance indicators across groups solely to the tests, without worrying about potential noise generated during sample selection.
This is not a trivial exercise. Depending on their research objectives, companies can tackle it using one of the five different sample-selection strategies we’ve identified:
- Pure random sampling
- Stratified random sampling
- Group balancing
- One-to-one matching
- Group matching
The strategies are based on the following criteria:
- The need to match a specific target group;
- The need to create segment-level insights;
- Group size; and
- If matching a specific target group, whether that group contains a single subject or multiple subjects.
Figure 1 shows the decision tree for selecting a strategy. Here’s how it works.
Use this decision tree to choose a sample-selection strategy
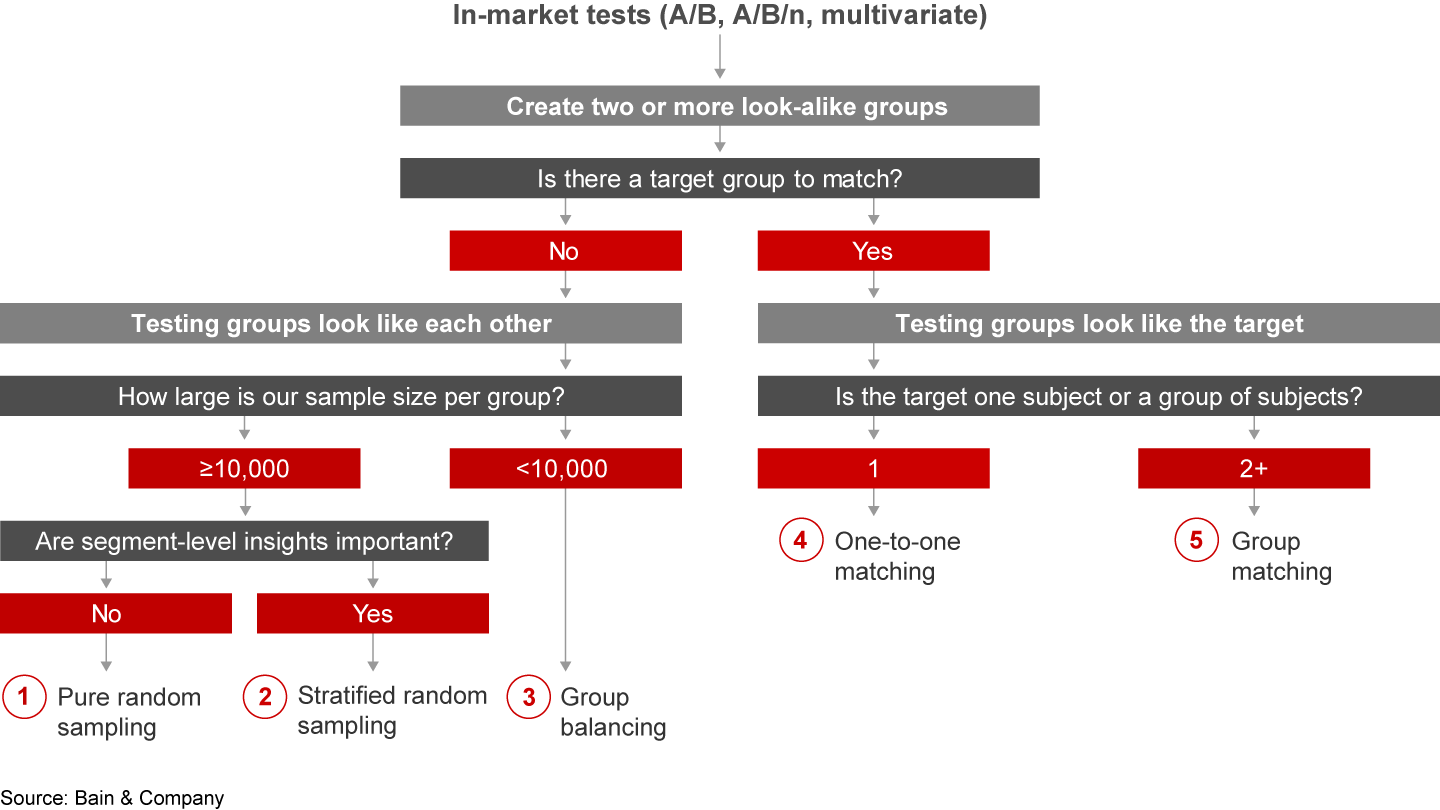
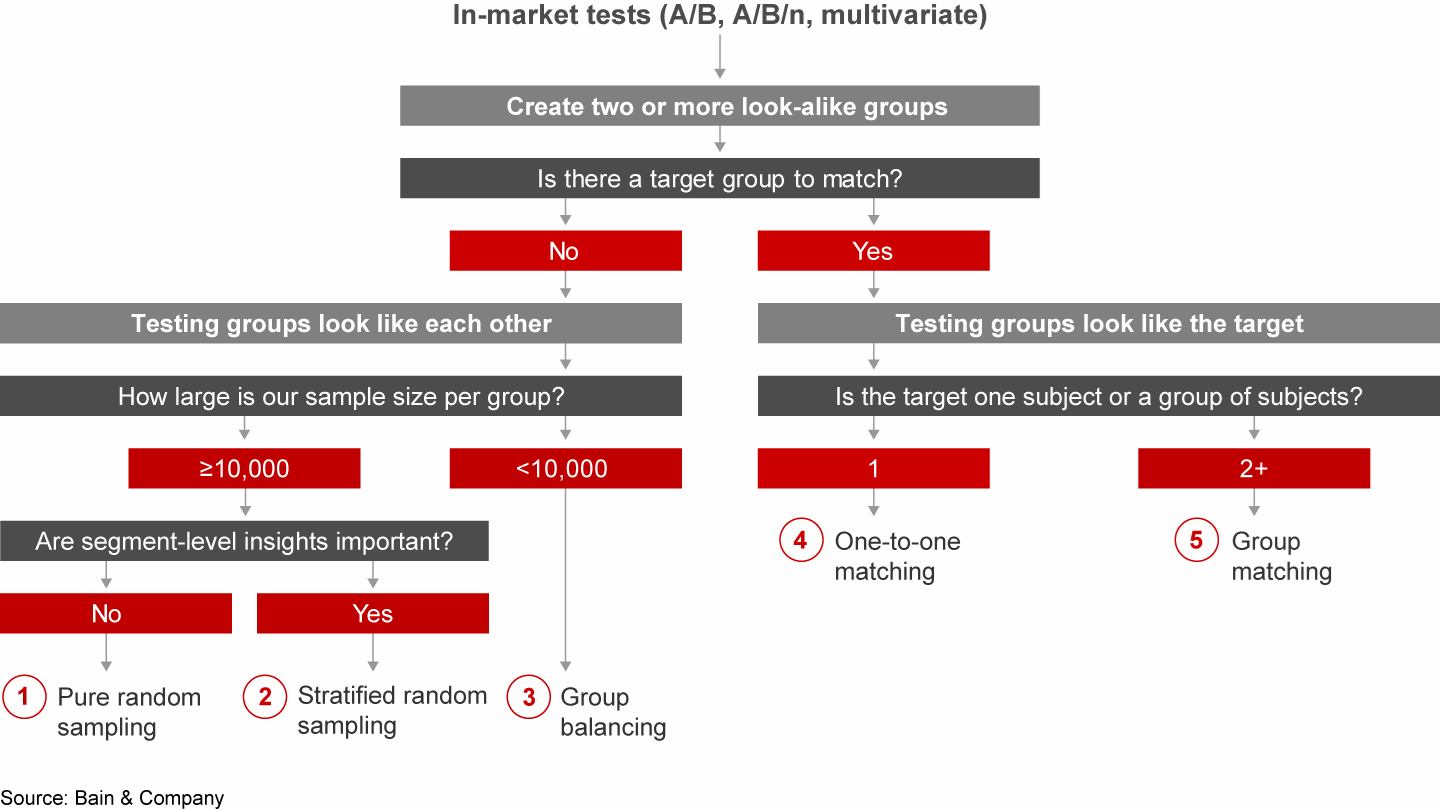
Without a specific target group to match, which is common for consumer markets, sample selection aims to divide the available target audience into multiple groups that look like each other. From there, you can use one of the following two random sampling strategies if the group size is large enough (typically exceeding 10,000).
Strategy 1: Pure random sampling
Pure random sampling works well if you’re not concerned about segment-level insights but instead care only about insights for the entire population. This approach randomly assigns subjects from the available target audience to one of the testing groups.
Strategy 2: Stratified random sampling
Stratified random sampling works well if one of your objectives is to obtain segment-level insights, such as which product should be offered to segment A and which to segment B. This approach creates a stratum at the segment level; then, within each stratum, it randomly assigns the research subject to one of the testing groups. As a result, segment distributions are the same across all testing groups and for the overall population. This feature allows you to identify the overall winner as well as segment-specific winners.
Strategy 3: Group balancing
A different situation arises when a company has no specific target to match and wants to test with relatively small group sizes (usually fewer than 10,000). Store tests, for instance, typically use samples of fewer than a hundred. Random sampling wouldn’t work because the group variations would be quite large. You need a strategy that balances all key characteristics across groups.
Group balancing employs an optimization algorithm designed to minimize the sum of group distance (the distance between each group and the overall population), subject to constraints such as the number of groups, group size (minimum and maximum) and whether or not any leftover is allowed (not all subjects are assigned). When we test only a couple hundred or fewer, group balancing vastly outperforms random sampling strategies.
Now let’s consider a situation where you have a specific target to match, which is more common for market testing or store testing. Here, the objective is to select groups of markets or stores that match the target, to minimize the distance of candidates from the target. Depending on whether the target has a single subject or multiple subjects, you should use one of the following two matching strategies.
Strategy 4: One-to-one matching
If the target has a single subject, such as a designated market area (DMA), and you want to find another DMA very similar to it, one-to-one matching involves a simple sorting exercise. You only need to calculate the distance of each potential candidate DMA to the target DMA and identify the single DMA that minimizes this distance.
Strategy 5: Group matching
If the target has multiple subjects, such as call centers or store locations, and you want to find a group of other call centers or stores that resemble the target, group matching involves an optimization exercise. You want the average of the new group to match that of the existing group, without matching each individual subject within the group. Because the size of each group usually is not the same, you need an optimization algorithm to determine the right size for each group, which subject should be chosen and how to assign the subject to a group. The solution is not unique, but you need to search in an intelligent way to find an answer that’s practical and good enough for your research objective.
These five sample-selection strategies cover the majority of situations involving in-market tests among a fixed target population. In our experience, the five strategies, when applied correctly, isolate true test effects from potential sampling biases. They allow a company to test successfully when sample sizes or detectable differences are small.
June Wu is a senior expert in Bain & Company’s Advanced Analytics practice. She is based in Boston.
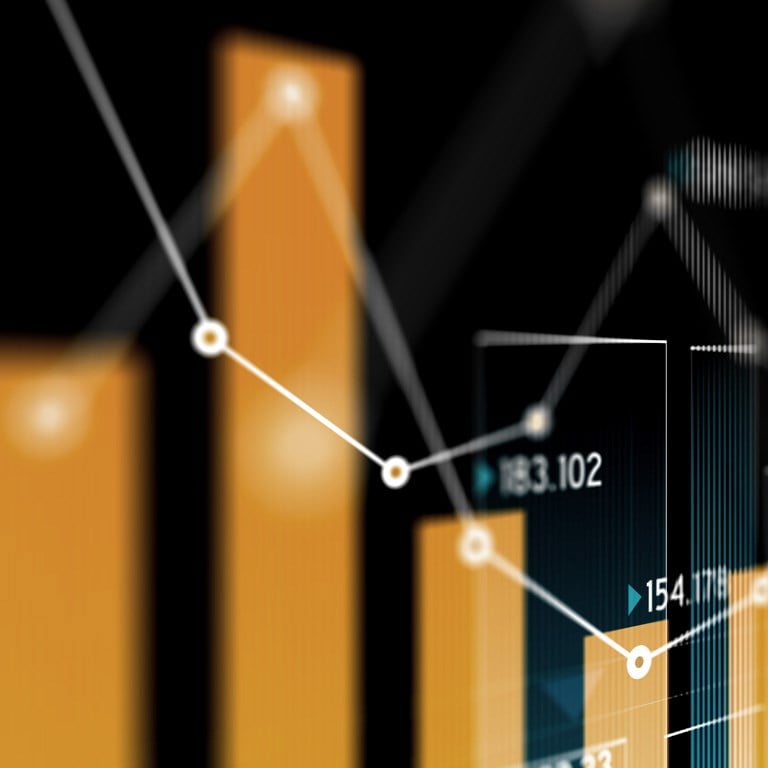
Advanced Analytics Expert Commentary
Success with advanced analytics requires both technical know-how and a thoughtful approach. In this series, Bain's experts offer practical advice on some of the most common data issues.