Global Machinery & Equipment Report
At a Glance
- 75% of advanced manufacturing companies say that adopting technologies such as artificial intelligence (AI) is their top engineering and R&D priority.
- AI is already improving productivity across the value chain, including in procurement, maintenance, and logistics.
- Scaling AI, including machine learning use cases, to generate value is a top priority for 78% of executives across industries.
This article is part of Bain's Global Machinery & Equipment Report 2024
As machinery and equipment companies build new tech muscle, they are investing heavily in artificial intelligence (AI). In fact, the AI market in industrial machinery, which includes intelligent hardware, software, and services, is expected to reach $5.46 billion in 2028, according to the Business Research Company.
Why? From supply chain volatility to cost pressures to the shortage of skilled workers, AI can help address top challenges facing machinery and equipment executives.
Many machinery executives increasingly see AI adoption as an urgent task. In the broader advanced manufacturing industry, 75% of executives say that adopting emerging technologies such as AI is their top priority in engineering and R&D, according to Bain research. Yet, while many companies have collected a mountain of data, a basic enabler of AI, most are not using it.
Leading advanced machinery companies offer a clue to success. Before investing in AI, they identify their core business challenges and how AI can help them improve processes and overall performance. That includes evaluating how specific types of AI, such as machine learning (ML) or generative AI, use data to create value. Early movers are using AI to solve key problems in procurement, assembly, maintenance, quality control, and warehouse logistics.
Some forward thinkers are beginning to deploy generative AI to synthesize huge volumes of unstructured data in order to revolutionize knowledge work, such as retrieving and summarizing relevant information from across the enterprise to answer questions from employees. Others are experimenting with generative AI service bots that partner with field technicians, for instance, to recognize more quickly when maintenance is required and to improve the quality of that work.
Those who are pulling ahead are also integrating AI solutions into processes and back-end systems.
How Can AI Be Used in Machinery & Equipment?
Explore the use cases with the highest potential.
The opportunity
Artificial intelligence is a broad term that encompasses technologies such as basic data analytics, ML, deep learning, and generative AI. Winning companies start by identifying their top business challenges and then selecting the specific AI solutions best suited to solve their unique key issues.
Ongoing disruptions such as Covid-19 and geopolitical instability have forced organizations to improve supply chain resilience and sustainability. The challenge is moving beyond reacting to problems after they happen. AI, however, can report supply chain bottlenecks in real time, predict potential disruptions in advance, and enable proactive planning to mitigate impacts to supply chains from an end-to-end business perspective.
AI can also track employee productivity and measure costs across all levels. AI helps companies shift their business models from simply selling machinery to offering machinery as a service, in which after-sales support and maintenance become part of the core offering. This includes applying ML to predict when equipment or parts need replacement, thereby reducing unplanned production downtime.
Finding qualified workers remains a challenge across the industry, especially for more complex engineering tasks. AI provides workers with information and insights to free them to focus on activities that add more value. It can also help train and upskill new workers to quickly come up to speed.
Generative AI in manufacturing is in its infancy, but many believe it will transform the sector. Specifically, the large language models that underpin generative AI fundamentally change how people interact with systems and documents. Generative AI can surface hidden insights from unstructured data that can lead to dramatic improvements in productivity, customer service, and financial performance.
Prioritizing the right business problems to solve
More than 90% of machinery companies already collect and store production data, according to a recent Bain survey. But most do not know how to derive value from that data. One reason is a lack of understanding about where AI can deliver the greatest returns.
Front-runners are already using AI to solve a variety of supply chain challenges (from cutting costs in procurement to using predictive monitoring) to identify failures before they occur in industrial assets, equipment, and infrastructure. In short, AI enables many digital applications that are top of mind for the industry (see Figure 1).
Artificial intelligence–enabled applications in industrial manufacturing will span the supply chain over the next five years
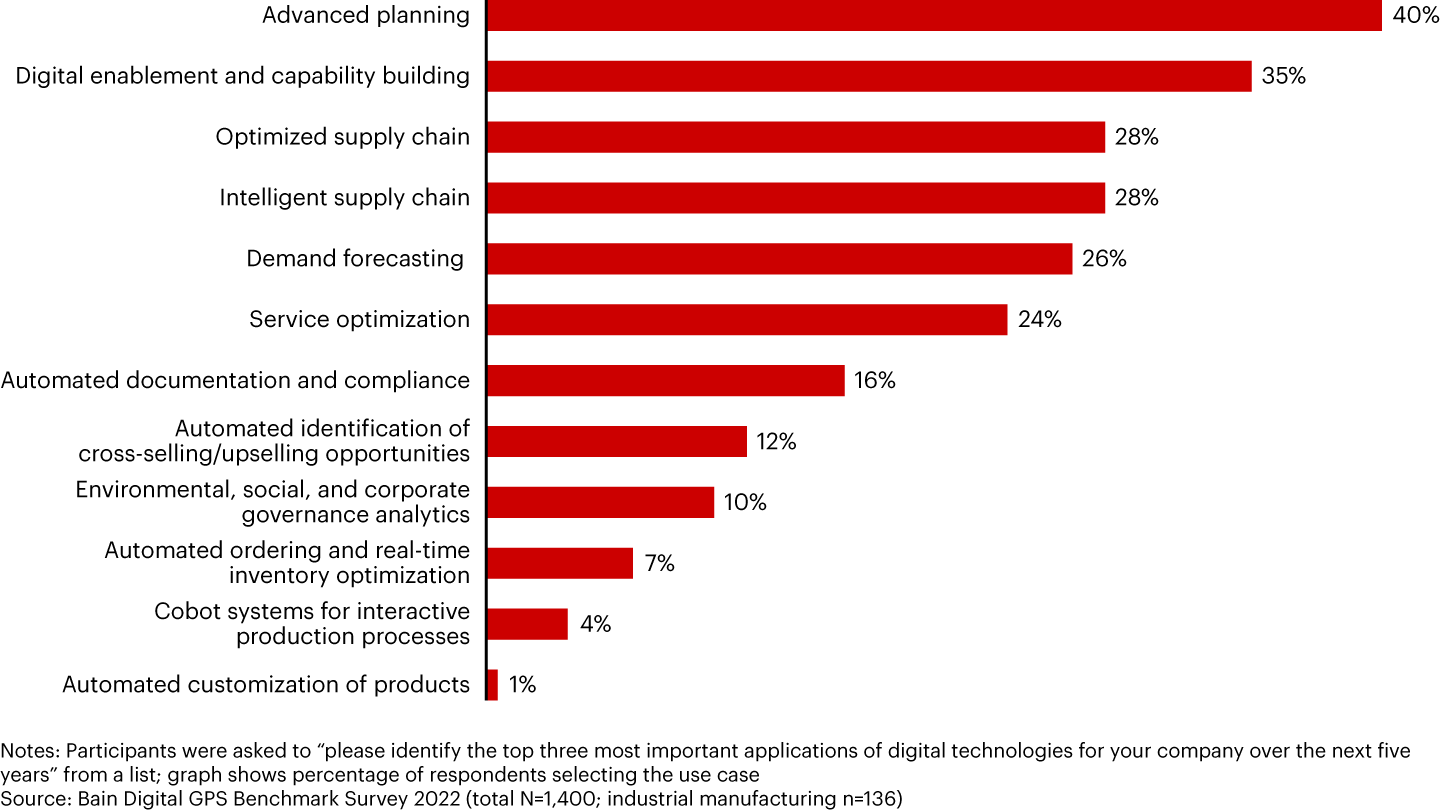
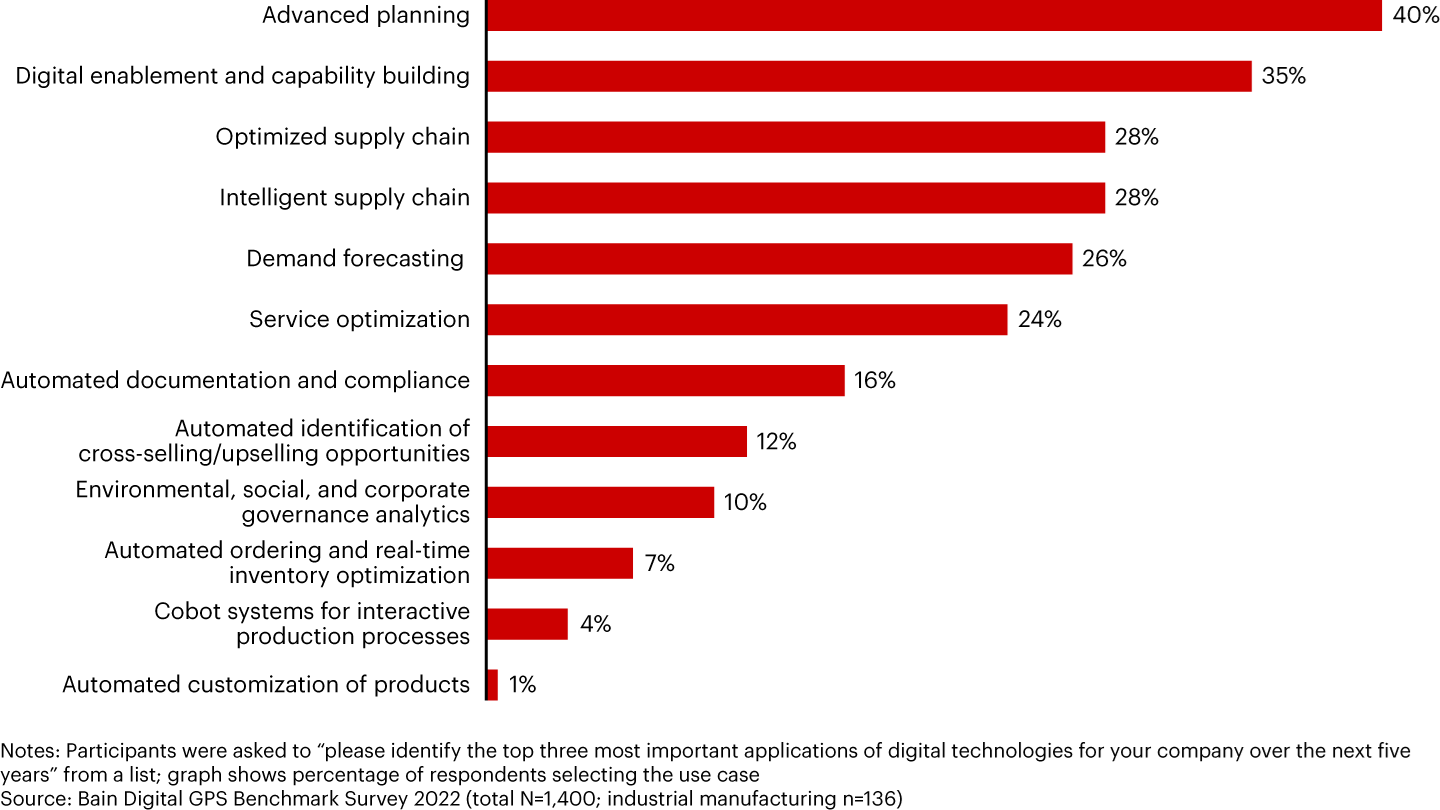
Three specific areas (of many) in which companies are cashing in on AI include minimizing assembly defects/improving quality control; boosting productivity; and streamlining warehouse management.
Minimizing assembly defects/improving quality control: AI can help identify mistakes in real time to improve assembly efficiency and product quality. For example, one machinery original equipment manufacturer (OEM) adopted AI-based video processing to track manual assembly activities, automate quality checks of manual assembly activities, and help optimize the use of resources and employees. Those solutions helped the machinery OEM reduce failures in the assembly process by as much as 70% while also cutting down efforts for quality checks by 50% for some lines.
In another case, a material supplier for machinery OEMs used computer vision to detect foreign objects in chemical bulk material instead of relying only on human inspections. The accuracy of the automated inspection increased by 80%, to greater than 99%, compared with today’s mainly manual visual inspection.
Boosting productivity: AI can also supercharge employee productivity, providing a boost to companies short on staff. One machinery manufacturer adopted an AI-powered industrial copilot that converts natural language into code and translates old programming languages into natural language, completing both tasks more expeditiously and at a higher quality than human developers. Among other benefits, engineers using this AI solution were approximately 5% more productive, according to preliminary results. Downtime costs also went down as there were fewer data deployment errors and issues were mitigated more quickly.
Streamlining warehouse management: AI can also help ensure that warehouses operate as efficiently as possible, meaning that they carry the appropriate items to meet demand and minimize extra inventory. One equipment machinery company, for instance, adopted an AI-based inventory management system that helped it minimize overstock while still fulfilling all orders.
AI also provides more flexible job production planning so that companies can allocate specific assembly activities to the most relevant assembly expert at a given time to maximize productivity. As a result, the manufacturer can simultaneously enhance the quality of its products and adjust processes to meet specific customer needs. In short, AI allows companies to customize and personalize without negatively affecting planning, productivity, and costs on the shop floor.
Navigating common AI obstacles
Scaling AI and taking successful AI pilots from one manufacturing line to other lines or other plants is not easy, but it is important. A 2022 survey by MIT Technology Review Insights showed that scaling AI use cases to generate value is the top priority for 78% of executives across industries (see Figure 2).
Artificial intelligence priorities for executives
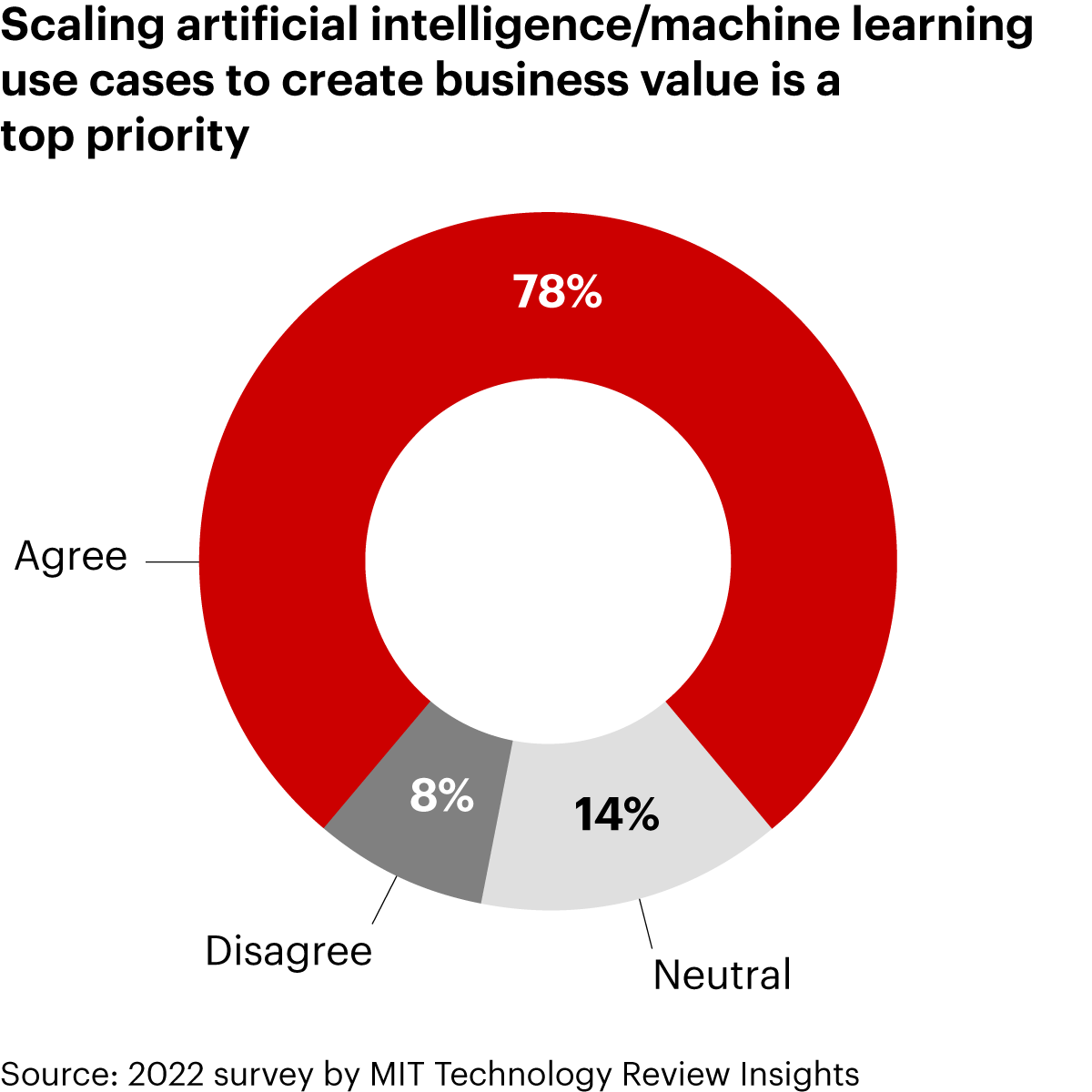
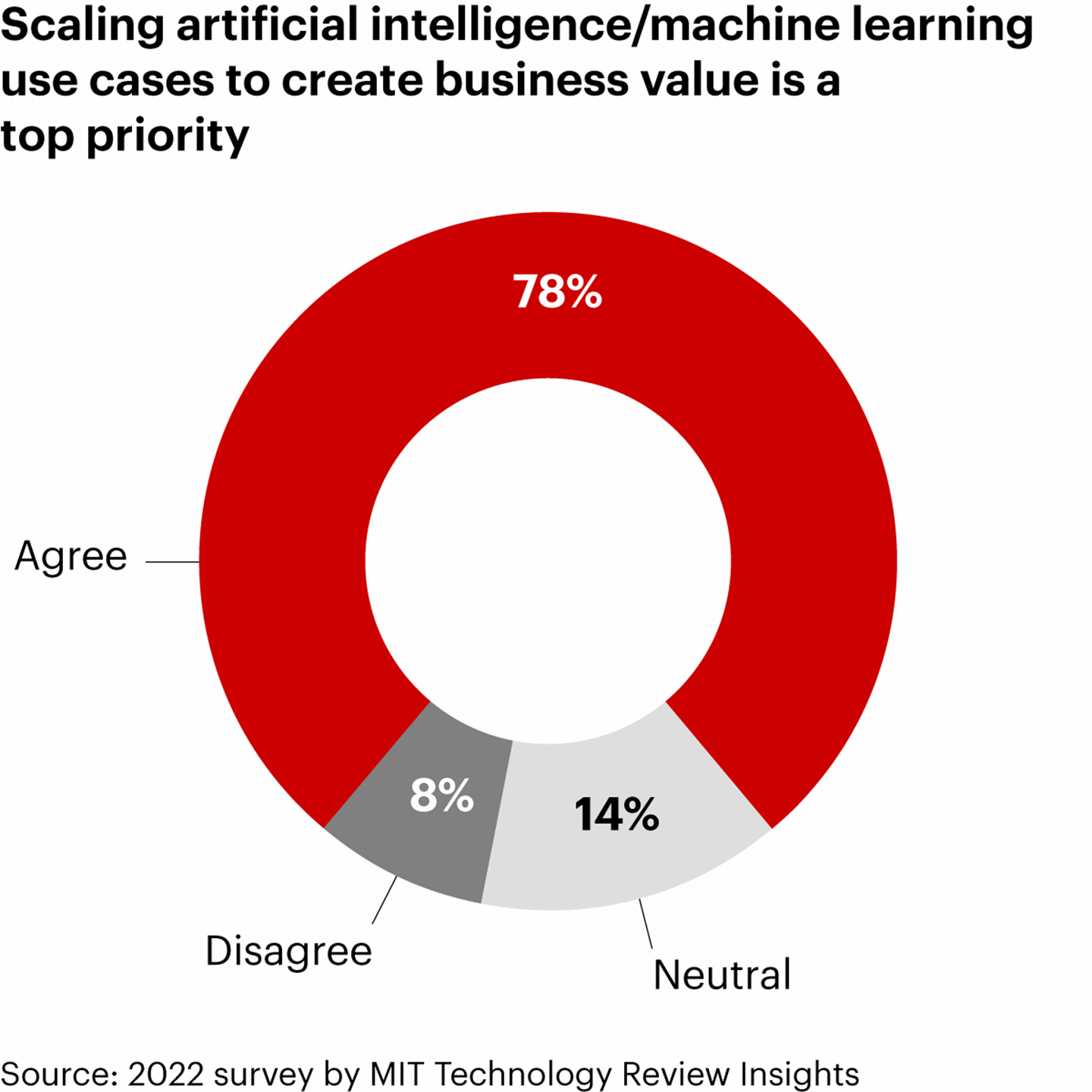
Top-performing companies monitor their return on investment throughout the AI implementation and ensure that they factor in all costs. While this may seem obvious, many companies forget to log computation costs on the cloud, for instance. Leaders also conduct regular governance checks (e.g., every quarter) to reassess their AI investment decisions.
Legacy software systems and fragmented data can also often pose problems as they create a chaotic data environment with low-quality data. The best teams standardize analytics systems and platforms to enable multiple AI use cases. They also use unified data models that allow them to merge many fragmented data sources into one.
To keep pace with rapid changes in AI, leaders use modular and loosely coupled components, connected via microservices, to make it easy to replace software. When integrating generative AI, they ensure that these new components enhance the existing data architecture. Successful companies also verify that efficient processes and tools (MLOps/DevOps) are factored into the technical architecture so that they can deploy AI at scale.
Leaders in AI also embrace a test-and-learn approach. Machinery engineers typically favor rigorous thinking and perfect product design. Software and AI work, however, require a test-and-learn, fail-fast approach using Agile methodology. In successful AI implementations, plant engineers and AI experts collaborate closely to create, test, and refine AI models until they meet the company’s goals.
Finally, machinery companies often struggle to find and retain employees with strong AI skills. To build in-house AI capability, many are bringing in external AI experts to train existing employees and increase data literacy throughout the entire workforce.
To retain skilled workers who may feel that some aspects of the work are uninteresting, successful companies have several approaches. Some are automating simple AI tasks so that experts can focus on more data- and analytics-intensive work. Others are developing expert squads to handle more complex AI use cases and crack data insight problems.
While each company faces different AI challenges, the leaders are addressing three core dimensions. First, they determine where AI unlocks the greatest value for the business. Second, they tailor the technology to address core problems and integrate it with their IT and operational technology setup. That means making sure that the technology is flexible so that it can be applied to immediate use cases but is also scalable in the future. Finally, they are developing a data culture that integrates AI skills and AI-enabled ways of working into the operating model.
AI has captured the imagination of machinery executives. As a growing number of companies experiment with and deploy new solutions, they are raising the industry bar for productivity and performance. Companies that defer investing will need to run twice as fast to keep pace.
The authors would like to express thanks to Josef Waltl, Kevin Denker, Robert Recknagel, Dennis Kuesters, Leonides De Ocampo, Marian Zoll, and Mary Stroncek for their contributions to this article.