Expert Commentary
At a Glance
- Understanding dependencies among drivers is critical when designing interventions.
- Two methods can achieve this understanding: dimensionality reduction methods and measuring variable uniqueness.
- Depending on a driver’s importance and uniqueness, each should be approached differently than other drivers.
When a company needs to determine which drivers have the greatest influence on an outcome, it can turn to key driver analysis. This allows decision makers to learn where to focus their efforts so as to achieve the greatest impact.
The Elements of Value in retail banking
In this commentary, we use the same dataset as in an earlier commentary. Our hypothetical retail bank is trying to understand what drives customer advocacy. Data comes from a survey of 2,500 consumers, asking how likely they are to recommend a certain brand to a friend or colleague—the core Net Promoter ScoreSM question. This likelihood to recommend becomes the outcome variable, the goal being to understand which variables have the strongest associations with the NPS® metric.
Potential drivers are a set of 30 attitudinal statements capturing how well a certain brand performs on the Elements of Value® as experienced by customers (see Figure 1). The survey asked respondents to rate their experience with the bank on each Element of Value using a scale of 0–10. Delivering on multiple Elements of Value can lift products or services above commodity status.
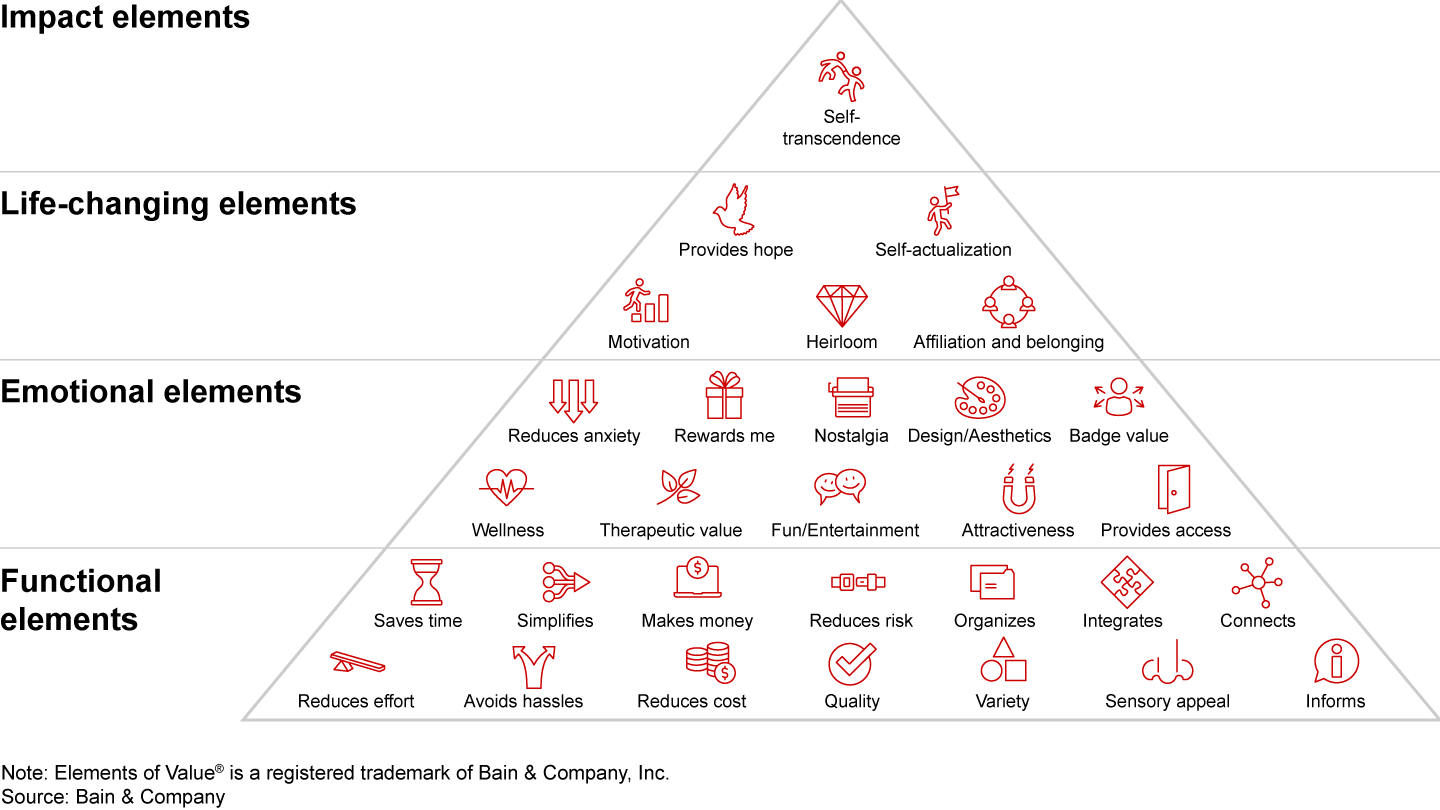
Variable importance
In the earlier commentary, we discussed analytical techniques commonly used for key driver analysis. We demonstrated that many methods, such as MLR, random forest variable importance, or partial correlations, penalize collinear drivers by reducing their ranking compared with other, less collinear drivers. This behavior can be problematic when collinearity among drivers is high. An important driver should still be important even if it is collinear with other drivers. We recommend using methods that assess the relationship of a driver with the outcome variable independently of other drivers in consideration. Correlation analysis is the most prominent of such methods.
The first task of driver analysis is to rank drivers in the order of their importance. We concluded the earlier commentary by showing the relative importance of the drivers according to Pearson correlation1 with likelihood to recommend (see Figure 2).
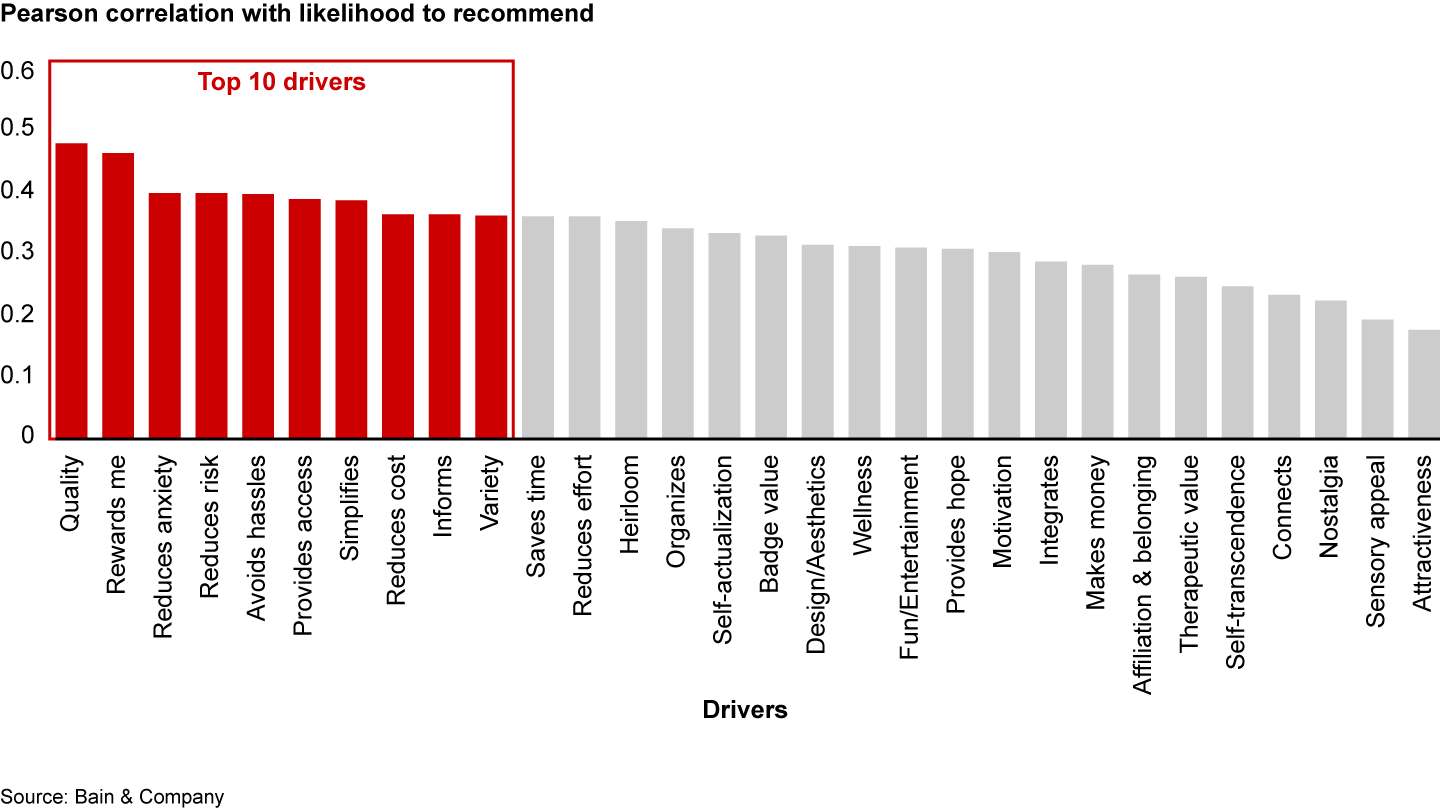
Quality was ranked as the most important driver, with Rewards me and Reduces anxiety in second and third place, respectively. This outcome makes intuitive sense given that our survey focused on retail banking products.
Many companies would conclude their driver analysis by shortlisting the top 5 or 10 drivers. We recommend taking an additional step, namely, analyzing how drivers are interrelated among themselves.
Understanding interdependencies among drivers
Understanding dependencies among drivers is not just a theoretical exercise. This analysis has practical implications that many business managers will find helpful when designing interventions:
- A driver that is independent from other drivers can be addressed on its own.
- When important drivers correlate with each other, it might be possible to address them all with a common approach.
An analyst can use one of two approaches, or both, to determine driver interdependences.
Approach 1. Dimensionality reduction methods such as principal component analysis (PCA). Dimensionality reduction can reveal groupings of drivers that are interrelated. As our drivers are psychometric measurements obtained through survey research, variable groupings in this context reveal drivers that are similar in the minds of survey respondents, and hence have similar ratings.
Figure 3 demonstrates the results of running PCA on our driver data, with the top 10 drivers highlighted by PCA groupings.2
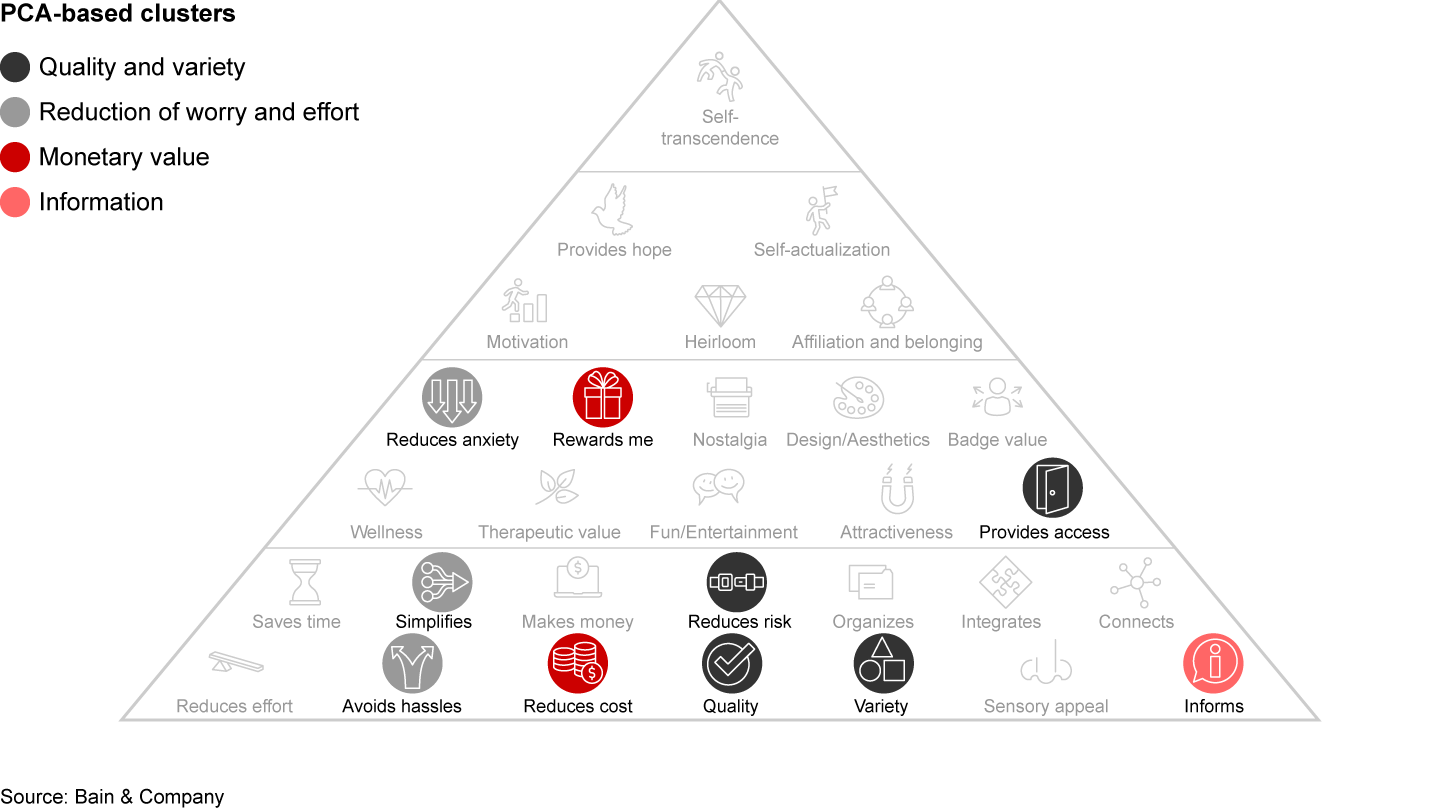
Drivers of the same color are interlinked in the minds of consumers. There are two potential interpretations:
- A single theme connects these drivers. In the blue cluster, for instance, one set of actions would likely improve perceptions of a brand on all elements in the group.
- A company that performs well on one driver tends to perform well on other drivers in that group. This likely occurs when there is no single theme in a group of drivers, yet they are linked together by PCA—such as Variety and Quality.
As with any descriptive analysis, PCA cannot directly answer causal questions. Yet the groupings can provide valuable clues. One might want to consider whether a grouping potentially contains a root cause driver, because improving that driver might boost customer perception of other elements in the group. In perceptions of Quality, Reduces risk and Provides access tend to move together in consumers’ minds. This might indicate that perceptions of quality of retail banking products are driven by how well a bank manages to reduce risk for customers and provide access to products and services. Quality can mean different things in different industries, so knowing what other factors Quality links to in an industry can be helpful.
Approach 2. Measuring variable uniqueness
To estimate variable uniqueness for a driver, we fit a random forest3 model where that driver itself is the dependent variable, and all other drivers are independent variables. R-squared4 from that model is an estimate of the percentage of variance in driver A explained by other drivers. To estimate variable uniqueness, we subtract R-squared from 1 (see Figure 4).
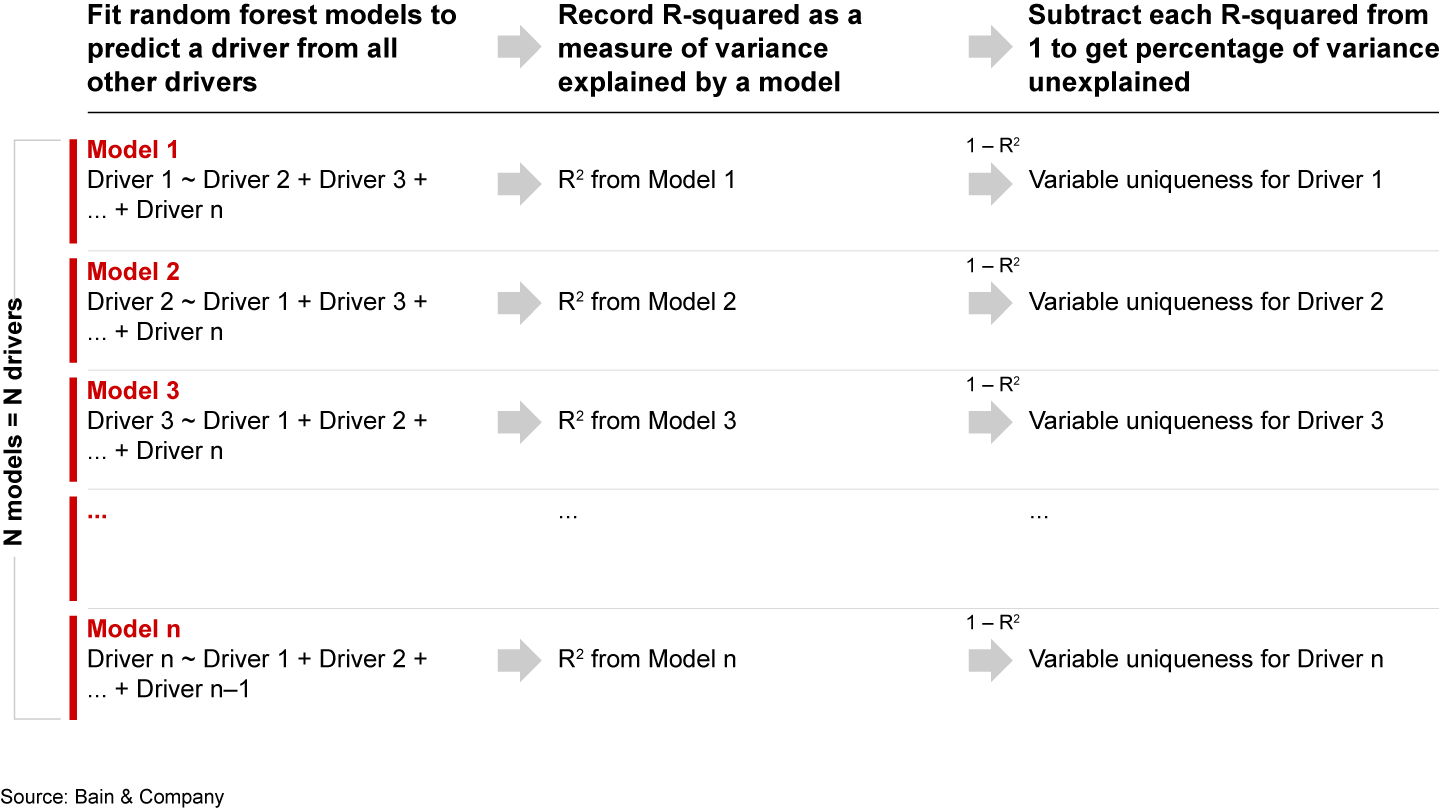
How can companies use variable uniqueness? We suggest using it alongside the chosen metric of variable importance. Figure 5 shows drivers plotted according to their importance and uniqueness, with a separate approach to drivers in each quadrant.
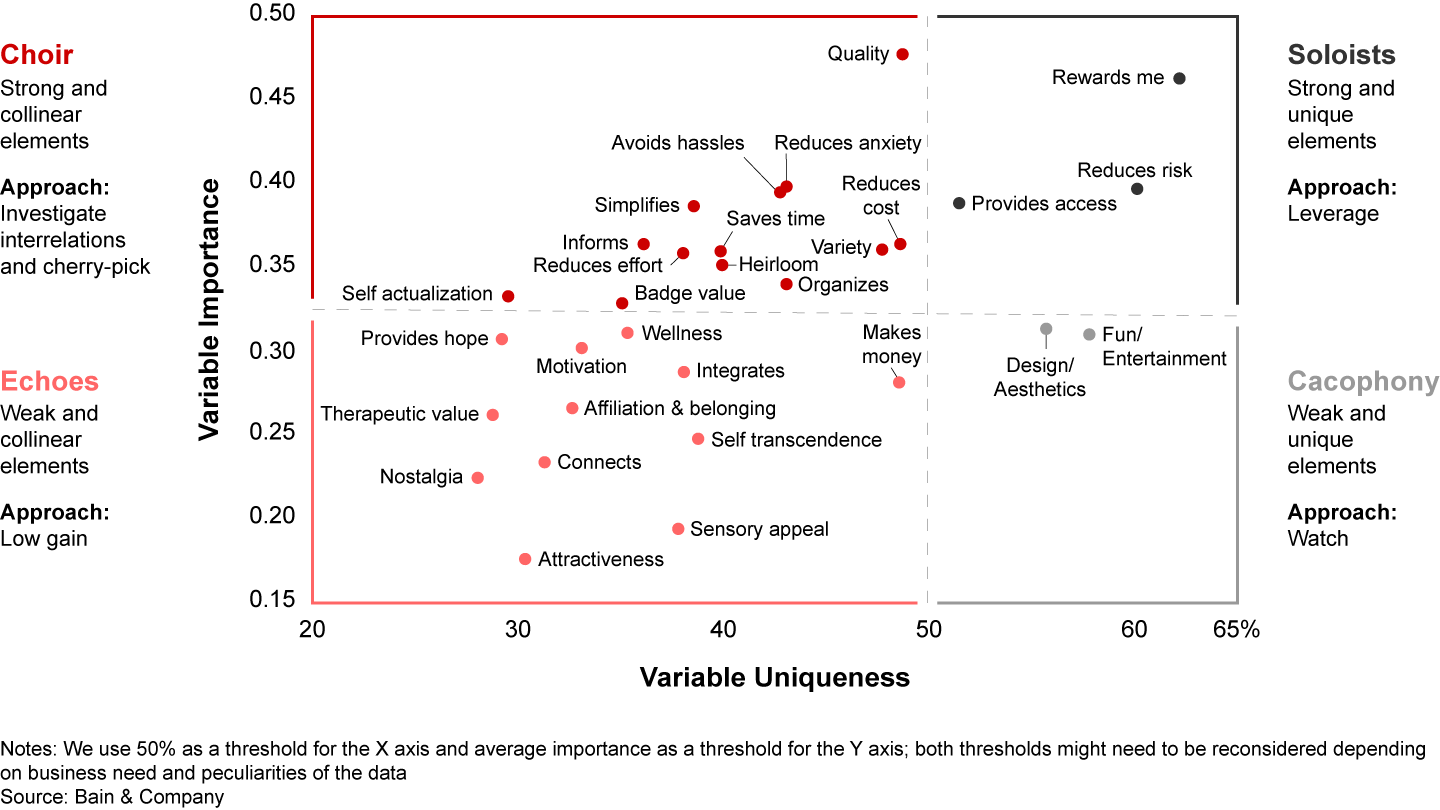
- Soloists: Drivers such as Rewards me and Reduces risk are strong predictors of the likelihood to recommend, independent from other drivers. Managers should consider creating a separate intervention for improving each of these drivers.
- Choir: Here, many important drivers have strong collinearity with other drivers. The right approach would be to pick a few to work on, as improving a few would likely improve consumers’ perception of the brand’s performance on other collinear drivers.
- Echoes: Many of these drivers are less relevant for retail banks. High collinearity can be explained by a halo effect, in which promoters of a brand tend to give nonzero scores to that brand even on metrics less relevant for the industry. A company would be better off focusing on drivers in other quadrants.
- Cacophony: These drivers are weak predictors, not collinear with other drivers. A potential explanation here is that some companies tried to differentiate on these metrics, but the drivers did not improve the likelihood to recommend among customers. A company might consider these drivers as additional differentiators once it has addressed the main levers of business outcomes.
An effective key driver analysis should include two steps. First, an analyst ranks potential drivers by the strength of their relationship with the outcome variable, using correlation analysis or other bivariate methods. Next, the analyst aims to understand interrelations among drivers. Understanding collinearity allows managers to make an informed decision on whether a single set of actions can address several drivers at once, or whether each driver needs an approach of its own.
Net Promoter®, NPS®, and the NPS-related emoticons are registered trademarks of Bain & Company, Inc., Satmetrix Systems, Inc., and Fred Reichheld. Net Promoter Score℠ and Net Promoter System℠ are service marks of Bain & Company, Inc., Satmetrix Systems, Inc., and Fred Reichheld.
Elements of Value® is a registered trademark of Bain & Company, Inc.