Expert Commentary
Doomsday has been postponed. With the introduction of the European Union’s General Data Protection Regulation (GDPR) in May, many analysts and businesspeople predicted chaos. However, the law has had only negligible consequences so far.
Some US websites were blocked for EU citizens because companies hadn’t prepared for the new regulation, which applies to non-EU companies serving EU customers. Just before the GDPR deadline, inboxes around the world were flooded with emails from companies asking for explicit consent to store personal user data for legitimate business use. For many customers, this was an opportunity to unsubscribe. And many companies used the introduction of GDPR to build (or rebuild) trust in their data usage processes.
Still, the analytics community remains broadly uncertain about what marketing scientists can do with customer data under the new regime. In general, modeling of personal data is still possible, but companies must apply strict anonymization standards and more stringently document their models.

Advanced Analytics Expert Commentary
Success with advanced analytics requires both technical know-how and a thoughtful approach. In this series, Bain's experts offer practical advice on some of the most common data issues.
Consider a common data-modeling situation affected by the new regulation: the linkage of primary research data with internal customer relationship management (CRM) systems. According to GDPR, survey data must be treated as personal data where it can be linked to an identifiable individual, meaning that survey responses and any additional matched variables will also be treated as personal data. In our recent work on this issue, we showed that it is possible to improve marketing models under GDPR using, for example, pseudonymized data, whereby a customer cannot be identified even if some of the individual’s database variables are linked to the survey data. (To make data pseudonymous, the linked variables are categorized before matching to the survey data—for instance, with rough spending ranges instead of precise amounts. In addition, a third party does the matching, so that neither client nor analytical service provider is able to link the survey data and customer database directly.)
A new take on predictive modeling
In the traditional approach, primary research data is linked with the CRM database on a highly aggregated level, such as by customer segments. Often, this is a good-enough solution. You can then use tagged segments to build a marketing strategy. However, aggregating the data causes the spicy taste of the sauce to fade. We wanted more granular detail that would yield greater value for marketing purposes. So we accepted the challenge to develop new approaches for linking survey data with CRM databases, in compliance with GDPR.
Typically, to link external and internal data, you first segment the survey data, with the number of segments ranging between 4 and 10. Then, you train a tagging model to link the segments by way of common attributes between the survey and the CRM system (see Figure 1). This meets privacy requirements, though with the trade-off of reducing variation in key survey measures.
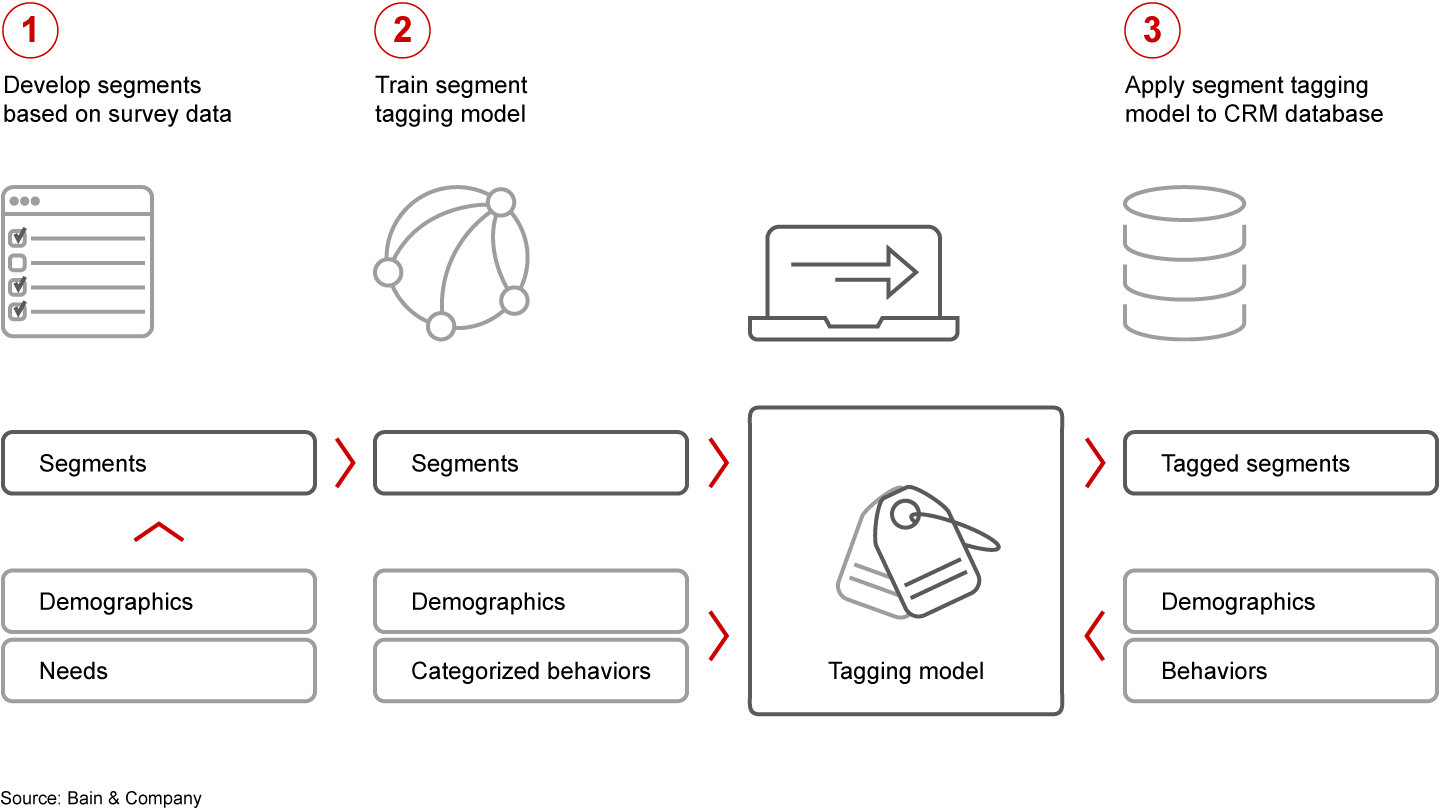
In our new approach, we took a cue from “record linkage,” a concept also used for segment tagging. Because segments are tagged to certain demographic and behavior profiles, why not match the original survey results instead of just the segments?
To accomplish this, we had to consider two factors. First, to meet the requirements of GDPR, we ensured anonymization, particularly by avoiding one-to-one matchings. Second, from a modeling perspective, we avoided overfitting. When aggregating by common attributes across the external and internal data, it is important to categorize the behavioral variables, which provides a first level of anonymization. In addition, we randomly perturbed some data to avoid overfitting and further strengthen data privacy. In situations where we didn’t match combinations of CRM attributes, these could be easily imputed (see Figure 2).
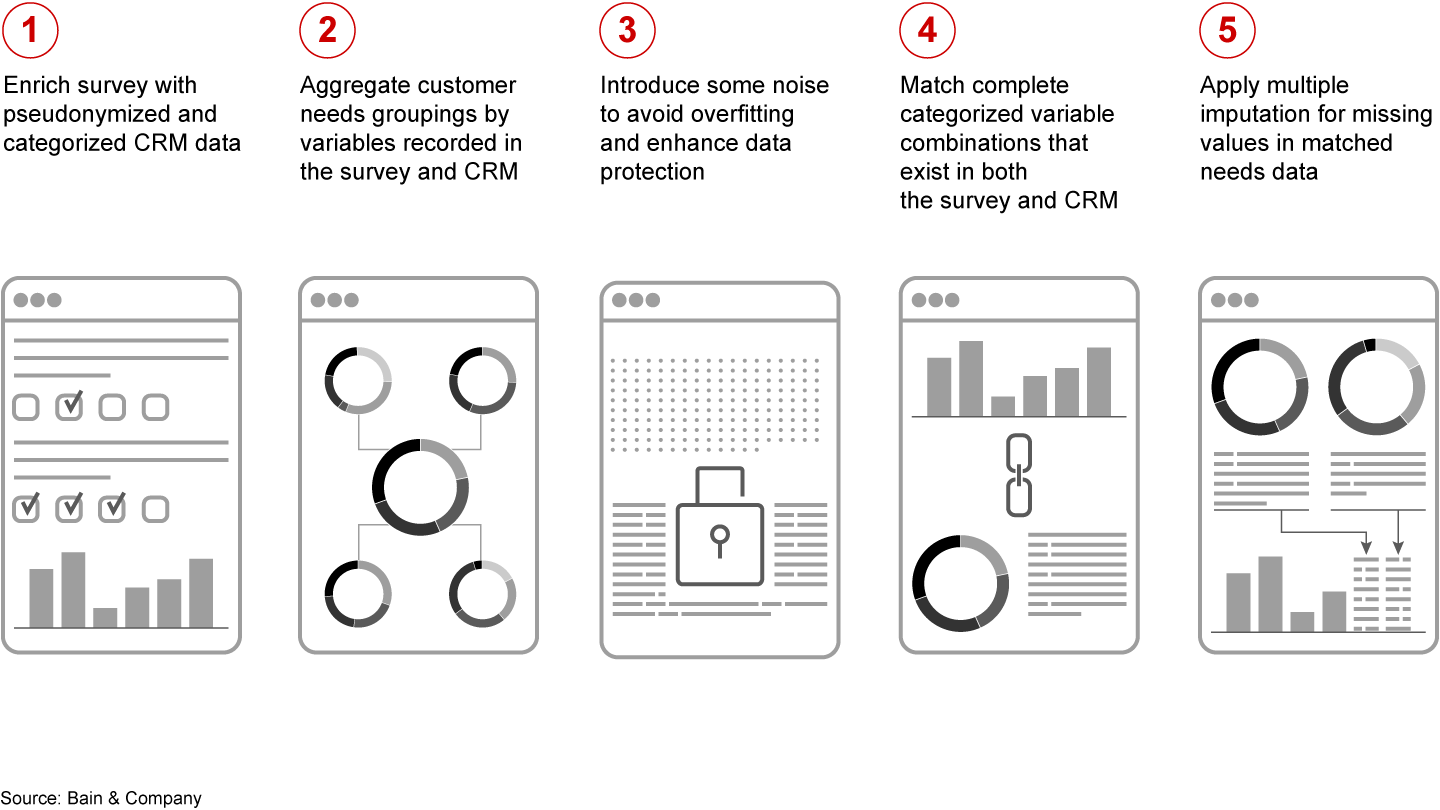
Alternatively, using probability matching as inspiration, we calculated a similarity score between the combinations in the internal and external data (see Figure 3). This score converts to a linkage weight and is used to calculate the weighted average of customer needs assigned in the CRM system.
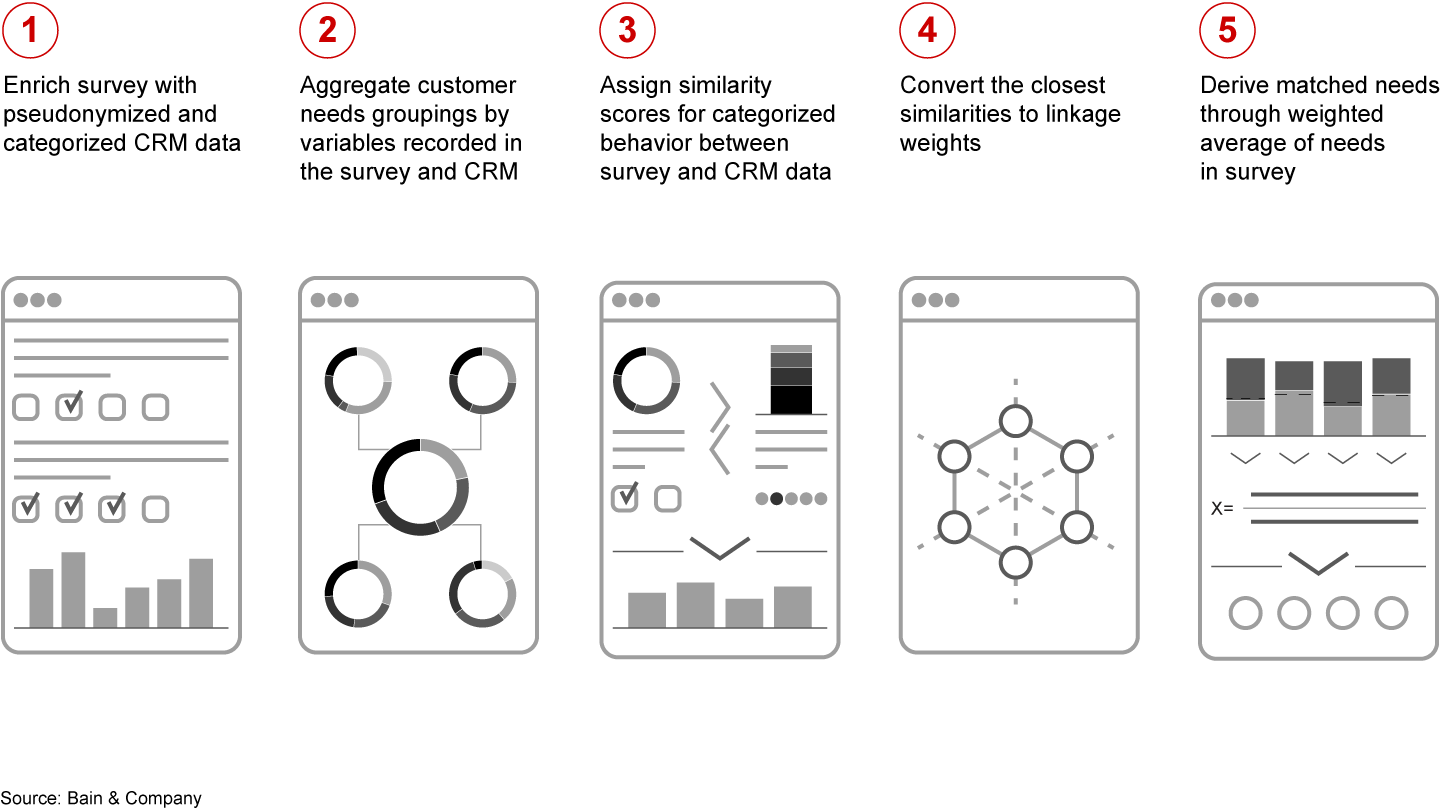
We have found that this new approach offers several advantages:
- It allows for simultaneous matching of many variables.
- It provides more variation and thus aligns more closely to the original data structure.
- More detailed and accurate data improves the efficiency of marketing applications and the underlying models, such as those for customer lifetime value, cross-sell/upsell and churn.
- It helps companies give customers more relevant product and service communications.
- Companies may see higher revenues from their marketing activities, at a lower cost.
- If needed for strategic marketing purposes, a company can develop segmentation based on the tagged data directly from the CRM database.
The method described here is one of many that can lead to strong data modeling under GDPR. If companies use the heightened focus on data privacy to improve their standards for modeling pipelines, then GDPR should not restrict them from developing innovative predictive models based on personal data.
Diane Berry and Josef Rieder are senior managers in Bain & Company’s Advanced Analytics Group. They are based, respectively, in London and Munich.