論説
概要
- Standard service-center and other customer service operations have run up against cost and performance ceilings.
- Predictive routing, powered by artificial intelligence, breaks through those constraints. Instead of a “first in, first out” queue, predictive routing makes a smarter customer/agent match for the interaction at hand.
- This approach combines an extensive customer profile with a rigorous assessment of the agent’s proficiencies, and accounts for the specific type of interaction.
- Predictive routing has increased customer loyalty and employee efficiency, reduced churn and handle times, and improved resolutions on the first contact.
The science of service centers has advanced with hold-time estimates, call-back options and voice-recognition technologies. Yet once the customer reaches an agent, odds are high that the agent will not be able to solve the problem in one go. Unsolved problems lead to more complaints, greater customer churn and wasted time of employees trying to calm upset customers.
Artificial intelligence (AI) promises to substantially improve the experience. Early efforts are helping companies improve the overall customer experience, while reducing costs—in staff time, service escalations such as field technician visits, and defecting customers—in the bargain.
AI-driven technologies form the engine for predictive routing, which pairs customers directly with agents across many channels, not through a traditional “first in, first out” queue, but instead on the basis of the customer’s individual characteristics and history, combined with a rigorous analytical assessment of each agent’s profile. For many companies, predictive routing is one of the most fruitful ways to employ AI right now. The technology is relatively quick and easy to deploy, and it generates results rapidly.
Companies with massive service operations—big banks, insurance carriers, healthcare providers, telecommunications carriers, technology providers—that have employed predictive routing in the past year or two in different types of work have begun to see such benefits as increased customer loyalty and lower churn, higher employee efficiency, reduced handle times and more problems resolved in the first contact (see Figure 1). For example, using predictive routing, Canadian communications and media company Rogers Communications reduced average handle time by 7% and increased customer retention by almost 3%. A mobile telecom operator in Western Europe saw its first-contact resolution rise by nearly 4% and its Net Promoter Score® (a key metric of customer loyalty) rise by four points.
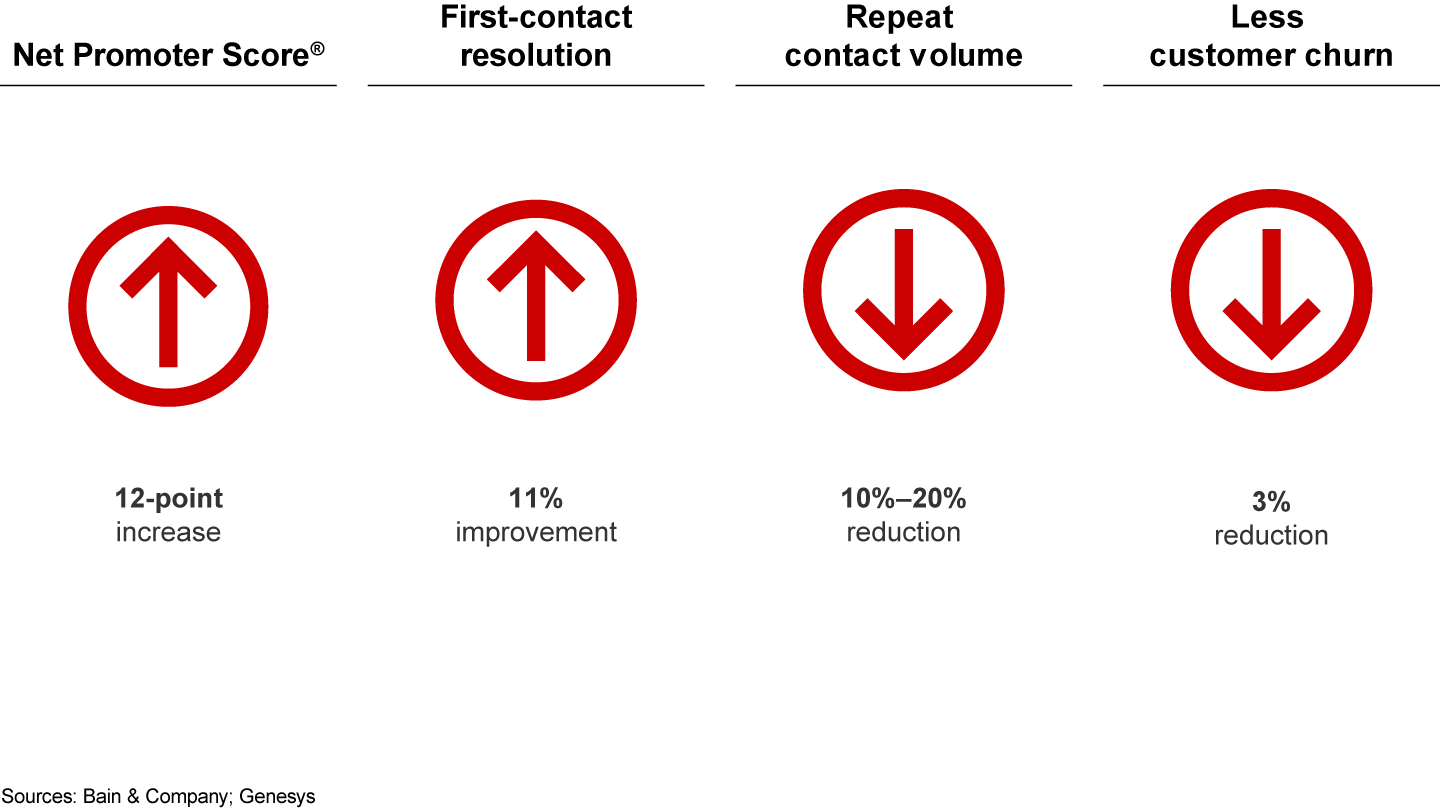
Over time, predictive routing will move beyond calls to match customers with agents through mobile applications, live online chat and asynchronous messages. Yet installing the technology is just the start to realize the full potential of predictive routing. Success also enables further improvements to service operations and behaviors, notably in employee selection, training and scheduling.
A perfect match
Consider the ideal service experience from a customer’s point of view. Sofia works from home and relies on her broadband Internet connection. She discovers the connection has broken and calls her provider, telling the automated answering system what happened. After a short wait, her call goes to an agent, Alexander. While Sofia remains on the line, Alexander remotely diagnoses and fixes the problem to get the connection back up and running. Alexander also notices that Sofia often exceeds her data allowance and offers her a suitable upgrade plan, which she accepts.
The interactions leave Sofia pleased on several counts, and Alexander feels good about helping her. His managers, meanwhile, appreciate the positive customer loyalty scores and the plan upgrade, while they have held down costs by avoiding multiple contacts or a field technician visit.
Of course, accomplishing this scenario is a big challenge. You have to ensure that the customer connects with a proficient agent, which is a more difficult feat than responding quickly and managing caller queues to the levels specified by a contract. Indeed, senior management increasingly wants service centers to help improve business outcomes such as sales, retention and customer advocacy. To do so means keeping track of constantly changing data on agents and customers; developing performance-based skill profiles, proficiencies and rules; and optimizing the ability to resolve an inquiry at the moment of need, within the customer’s patience threshold.
Bain Partner Jeff Melton describes how AI and automation can help customer service agents solve customer problems more efficiently.
AI maps out a better route
Predictive routing applies AI to historical data and real-time context in order to identify factors that influence each interaction. These factors could be a customer’s recent purchases, transactions and service requests. For instance, the technology sees that Customer A contacted the company twice in the past week about a bill, or it scours external web-search data to see that Customer B visited home sale and mortgage-comparison sites.
The AI engine compares customer profiles and agent profiles with the eventual outcomes of the interactions in similar situations—outcomes ranging from sales conversion to the Net Promoter Score assigned by the customer. That information goes into a machine-learning algorithm that predicts a preferred match of customer and agent, based on the outcome desired. The routing engine then applies the preferred customer/agent match, given the constraints of the moment such as service level and agent availability (see Figure 2).
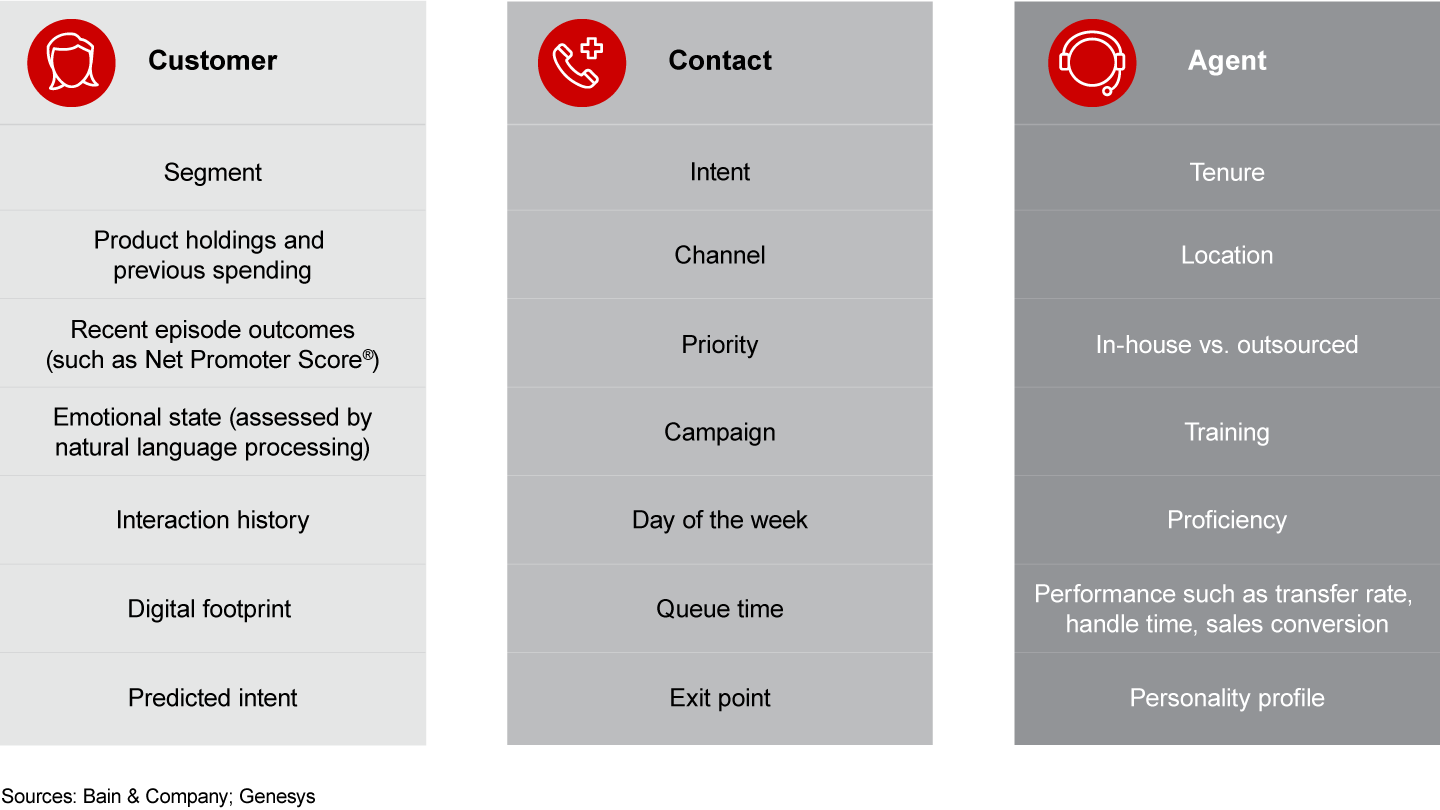
Here, AI can rectify a fundamental flaw in service-center organizations today: reliance on the agent’s profile rather than demonstrated proficiency. Many organizations keep spreadsheets populated by agents’ education, certification and presumed proficiency based on training and tenure, none of which accurately predicts how well someone can handle interactions of certain types or customers with certain profiles. A large variation typically exists among agents who have similar skills on paper. Current skills tables often have little to no correlation with actual business outcomes, because the correlation typically gets calculated at an aggregate level, not an individual customer, interaction, agent or outcome level. Routing based on traditional operational metrics therefore tends to have a low probability of business success. This process delivers broad-brush pairings, with unsatisfactory outcomes for both the business and the individual, which ultimately can cost millions in forgone value each year.
Predictive routing engines, by contrast, automate the process of characterizing each agent according to his or her ability to handle various types of contacts, and each customer for his or her value to the company, outcome data and interaction history. The engines use external databases and algorithms to fill out the broader context for the interaction. And machine learning techniques now can flag behavioral patterns in order to continuously improve the algorithms that predict and prescribe the optimal outcomes. For instance, multiple contacts by a customer near her contract-renewal date may signal a propensity to cancel a contract; a call after a recent bill could mean the customer is disputing a charge; or the first inquiry after a bank sends a credit card to a customer likely will be to activate the card.
The predictive routing approach also reduces transfer activity through better customer/agent matches. Companies typically direct agents to avoid transferring interactions, so when an agent receives an interaction he isn't confident to handle, he feels stress. Predictive routing reduces the incidence of this problem by increasing the quality of the match (see Figure 3). And when a call is transferred, it is more likely to reach an agent primed to help.
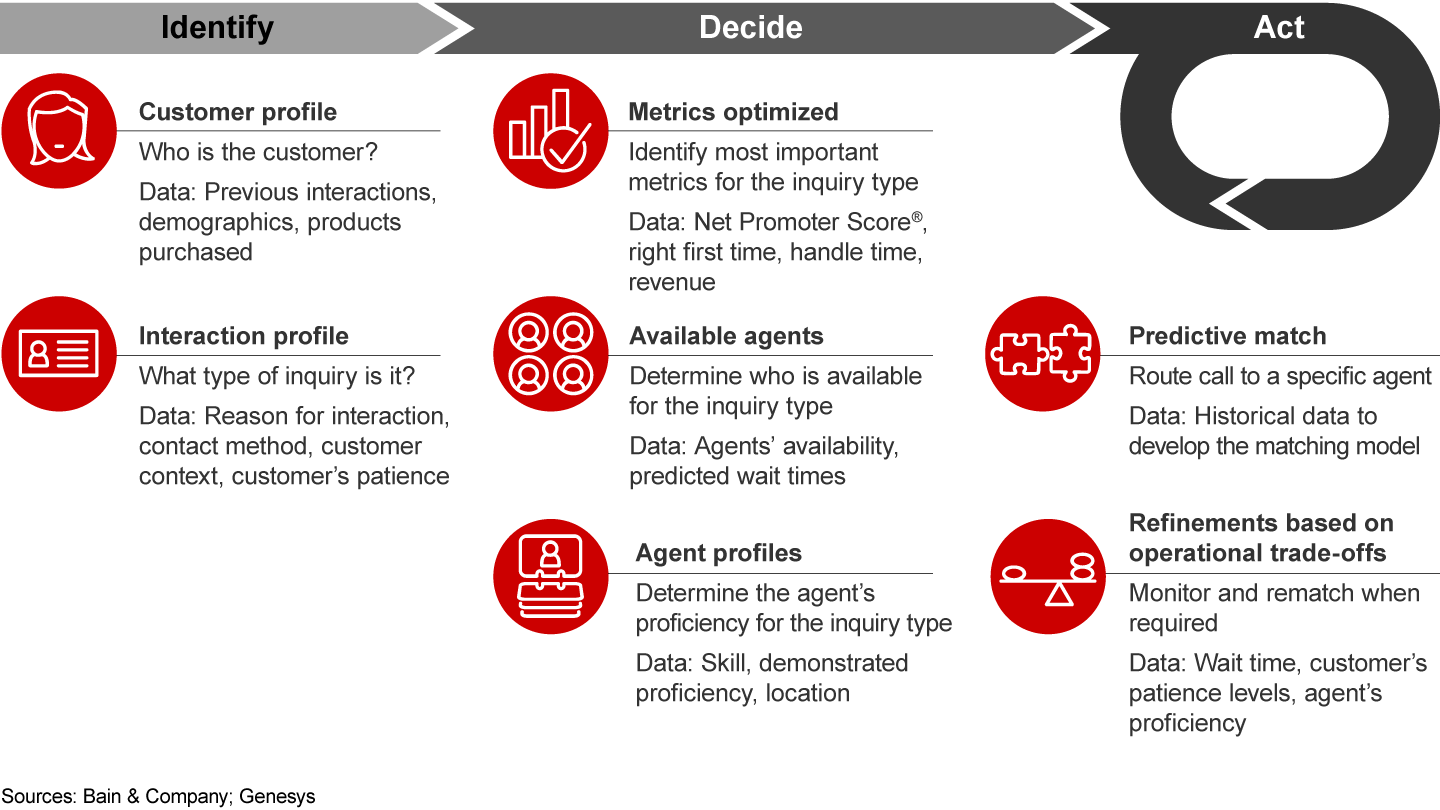
Time still equals money, but not the way you thought
Predictive routing turns the traditional consideration of time on its head. Companies often use customer wait time as a metric for service success—say, a target of answering 80% of calls within 30 seconds.
But many customers are more willing to wait for good service than companies realize. They want to connect with an agent proficient in resolving their enquiry, within a reasonable threshold. How much waiting will people tolerate? You can determine patience levels by calculating the period of wait time at which the Net Promoter Score for the interaction, or another business outcome, begins to decline. Analysis by Bain and Genesys of the correlation between Net Promoter Score and wait-time curves shows that patience levels vary by country, the reason for the inquiry, the channel and how a company positions its service. In general, though, patience levels are often higher than managers typically believe (see Figure 4).
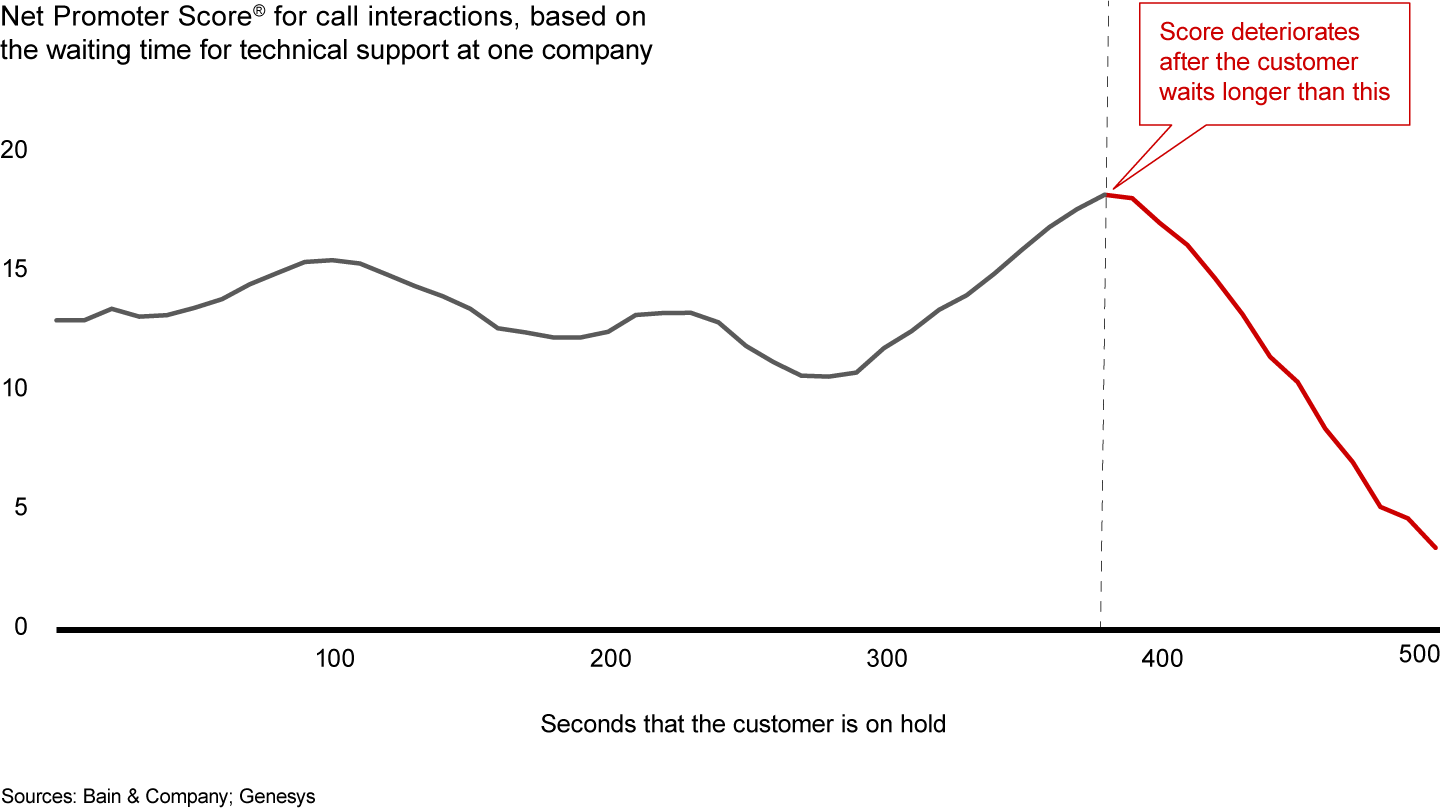
Taking the most granular view—the type of inquiry informed by the context of the particular customer—helps companies respond more appropriately to each inquiry. And with more flexibility in wait time, companies can steer the inquiry to the best-equipped agent. Companies using patience-level and empirical-proficiency metrics have significantly lifted first-contact resolution and Net Promoter Score, as agents spend more time on the issues they are most suited for. By understanding patience levels and the likely value of an interaction, companies can design wait time to increase the certainty of a high-quality match between customer and agent.
Choosing the right data and the right problem
Companies have collected valuable data in their service centers for years. Now they can use the data to predictively route inquiries based on real-time, performance-based proficiency. To assess the opportunity, executives can ask a few key questions:
- Do we use interaction outcomes to define agent proficiency, and do we use agent proficiency to route contacts?
- What share of our contacts land with agents who are most proficient dealing with that type of contact?
- What share of agents' time is spent on contact types at which they are most proficient?
- How steep is our experience curve for each type?
If a company does not know the answers, or if the answers do not place a company among the best operators, executives probably have a sizable opportunity. A brief analysis will estimate the value of improving routing outcomes across the relevant channels, and then senior executives should identify their main goals in deploying the technology: Increase loyalty scores? Grow sales? Maximize first-call resolution? If the prize is big enough, it’s worth moving forward.
At that point, you need to find the right technology and a provider that has a proven history using AI, can demonstrate the quality of the outcome, and can get you up and running quickly. Considerations include the following:
- Value. Can the provider quantify the potential value and commit to delivering it?
- Automation. To what extent does AI automate your routing environment?
- Transparency. Can you see the decisions that AI makes to inform operational improvements, or is the technology a black box?
- Ease of use. How quickly can AI be deployed to prove value, and how quickly can it scale up across an enterprise?
- Flexibility. How does AI handle changes to business priorities and metrics such as Net Promoter Score, revenue or customer churn?
- Adaptability. How would the technology cope with disruptions to the current system?
- Cybersecurity. What measures exist to ensure safety and privacy of customer data?
Predictive routing will make the jobs of the front line and the service center easier. Over time, the new method of matching customer demand with agent supply may require improving how you manage agents and plan capacity. So managers should prepare to educate staff about how the new system works, and should adapt individual coaching to help each agent serve the newly tailored set of customers.
With the right planning and a skilled technology provider, companies that incorporate predictive routing into their service operations stand to seize an advantage over the next few years. They will be able to reduce costs, increase sales and saves, raise the level of customer advocacy, create more satisfying jobs and keep talented agents engaged with the tasks where they are most proficient.
Net Promoter Score®, Net Promoter System®, Net Promoter® and NPS® are registered trademarks of Bain & Company, Inc., Fred Reichheld and Satmetrix Systems, Inc.
Jeff Melton is the global leader of Bain & Company’s service design and operations work. Corrie Carrigan leads Bain's service-center work in the Americas. Nikola Glusac leads Bain's service-center work in Europe, the Middle East and Africa. Graeme Provan is a global solution director at Genesys. The authors are based, respectively, in Melbourne, Chicago, Berlin and San Francisco.